Our program today is maximizing the power of data with embedded analytics tools. I'll be talking about some trends around that are impacting the direction of embedded analytics. Some of the issues around increasing the potential of the best embedded analytics with cloud platforms and frameworks, which is really one of the big changes in this world of embedded analytics.
Then I will talk about monetization basically the idea of trying to use data and analytics for monetization purposes, and we'll get into that and what can be done with employees and so forth, to turn it around into kind of productizing it. Then I'll conclude with some recommendations.
I think that the big picture is digital transformation. That's kind of one of those buzz words out there, but it's no denying the fact that data is being generated everywhere. We have edge devices. We all are carrying around our mobile devices, and they are generating data about all kinds of things, our activities or behavior, and then of course, geo-location and security issues and things like that.
Then organizations are increasingly competing with software as a service and also a platform as a service, other Cloud based applications and data platforms and services. There's a lot of data moving to the Cloud being generated in the Cloud. Then there is the whole area of online customer behavior and consumer behavior, which stretches across multiple kinds of systems.
#1 Ranking: Read how InetSoft was rated #1 for user adoption in G2's user survey-based index |
|
Read More |
Rich Data to Analyze
It could be social media kinds of systems, but also the numerous kinds of websites and marketing campaigns that are out there that customers and consumers may be interacting with. There's a lot of rich data out there that organizations would like to get to. There are some frustrations about the separation between standalone data, data warehousing, and BI analytics kind of platforms. Then there are the business applications or process applications, transaction oriented applications and other applications that are interfacing with customers and consumers.
What we're really talking about here is trying to reduce that divide and bring data together so that organizations can reap faster value from all this data. That the old model of separated data is breaking down a bit because of the amount of data and the demand for it. It's right in line with what we're seeing in terms of trends all across industries, and so I have a little graphic here that we have just about transforming insights.
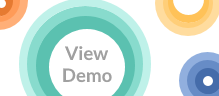 |
View a 2-minute demonstration of InetSoft's easy, agile, and robust BI software. |
Demand for Intelligent Data Processing
We see demand for intelligent data processing, so data processing itself, and trying to embed intelligence and AI into it. There is also automated model building for analytics, including natural language interfaces so that's a little bit more easy for users to work with instead of having to go to a separate system. Transferring business processes is really a little more of a focus of what we're talking about here.
Again, there are lots of things going on in terms of embedding machine learning and other kinds of AI models into business processes. Proactively companies are trying to make systems alive with the ability to provide recommendations, predictive insights things like that, so proactive alerting, proactive intervention, and automating that. In addition developers are transforming products, and we see products out there, of course, the voice assistants, digital assistants, talking machines, virtual assistants and then systems that can respond in that proactive way to situation awareness, situational awareness. Certainly the idea of embedding is right in line with what we're seeing a lot of areas in the industry.
An Example of AI Being Used in Proactive Intervention
One compelling example of AI being used in proactive intervention is in healthcare, specifically in the field of predictive analytics for patient care. Hospitals and healthcare systems are increasingly leveraging AI algorithms to analyze vast amounts of patient data, including electronic health records (EHRs), medical imaging, lab results, and vital signs, to identify patterns and trends that could signal potential health risks or adverse outcomes. By applying machine learning and predictive modeling techniques to this data, healthcare providers can proactively intervene to prevent or mitigate health issues before they escalate.
For instance, AI algorithms can analyze historical patient data to predict the likelihood of readmission within a certain timeframe for specific patient populations, such as those with chronic diseases or undergoing certain medical procedures. By identifying patients at high risk of readmission, healthcare providers can implement targeted interventions, such as personalized care plans, medication adjustments, or follow-up appointments, to prevent readmissions and improve patient outcomes. These proactive interventions not only enhance patient care but also reduce healthcare costs associated with avoidable hospitalizations and complications.
Furthermore, AI-powered decision support systems can analyze real-time patient data streams to detect early warning signs of deteriorating health or medical emergencies, such as sepsis or cardiac arrest. By continuously monitoring patients' vital signs, symptoms, and physiological parameters, these systems can alert healthcare providers to potential issues before they become critical, enabling timely intervention and potentially life-saving treatments. By integrating AI-driven predictive analytics into clinical workflows, healthcare providers can deliver more proactive, personalized, and effective care, ultimately improving patient outcomes and enhancing the overall quality of healthcare delivery.