Machine Learning Use Cases That Were Impossible Before
Below is the continuation of the transcript of a Webinar hosted by InetSoft on the topic of Enabling the Intelligent Enterprise with Machine Learning. The presenter is Abhishek Gupta, Product Manager at InetSoft.
Let's go to the other side of the business to speak about machine learning use cases that were impossible before. What if you could not just put marketing dollars in putting your brand or logo somewhere, what if you couldn't just sponsor a team or a particular venue or event but you could measure the impact and the outcome of that in real time.
Thanks to computer vision and full HD video processing we are able to find your logos, your products, your offerings in real time in commercial broadcast quality and video path and to determine accurate impact metrics so that you can measure what you pay for in terms of scholarship for advertising and make that available in near real time.
These are capabilities that have never been available on the market before, and this aims to revolutionize the way we do advertising impact metrics and return on advertising investment calculations. We look forward to deriving many additional visual and video base use cases that bring concrete business value to the enterprise.
It's interesting to see that machine learning touches basically every aspect of a business, whether it's sales or marketing, whether it's technology, whether it's operation or whether it's finance. Machine learning is and will be everywhere. You will see.
#1 Ranking: Read how InetSoft was rated #1 for user adoption in G2's user survey-based index |
|
Read More |
Innovative Machine Learning
This brings us basically to the next topic about some of the popular and innovative machine learning technology and applications that are being implemented today.
What we see is that devices that have natural language processing provide interactive responses to people and to other devices, and some examples are Amazon Echo, Google Home, Netflix and recommendation engines.
I see this myself as a very interesting development of machine learning particularly the natural language recognition parts. They give us an interactive customizable experience that we've never seen before. What we see is also machine learning has enabled the popular digital assistants.
We see all the applications that you have, whether it's Apple's Siri or some other speech recognition applications. They are responsive – yeah they provide responsive tools also via your mobile. It's predicted that machine learning could, let's say make text and maybe GUIs basically really obsolete in the future, which is interesting development to follow.
What we see as well is that machine learning applications are altering and revolutionizing various industries whether we are talking about medical research, and whether it's diagnostics and healthcare, improving consumer applications in all different kinds of industries. For example, machine learning can provide healthcare personnel with real time information about the patient's care or making diagnostics and treatments much more accurate, and they can use augmented intelligence to advise the doctors.
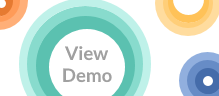 |
View a 2-minute demonstration of InetSoft's easy, agile, and robust BI software. |
In the customer service industry we see that machine learning can help them to offer relevant fast personalized experiences, and we see some substantial changes in this as well. What we also see is machine learning can classify and categorize social media posts, so everything in the marketing domain. It enables companies to deliver higher quality customer experiences through all the channels that you're connecting with people from a company perspective.
How Can ML Be Used in Jet Fuel Production?
Machine learning (ML) can be instrumental in optimizing various aspects of jet fuel production, enhancing efficiency, safety, and sustainability. Here's how ML can be applied in the context of jet fuel production:
-
Process Optimization: ML algorithms can analyze vast amounts of data collected from sensors, production logs, and historical records to optimize the jet fuel production process. By identifying patterns and correlations in data, ML models can suggest adjustments to operating parameters such as temperature, pressure, and flow rates to maximize yield and minimize energy consumption. ML-based predictive models can also anticipate equipment failures or process deviations, enabling proactive maintenance and minimizing downtime.
-
Quality Control: Ensuring the quality and consistency of jet fuel is essential for meeting industry standards and regulatory requirements. ML techniques, such as spectroscopy and chromatography, can be used to analyze the chemical composition of raw materials and finished products in real-time. ML models trained on historical data can predict the properties of jet fuel based on input variables such as feedstock composition and process conditions, enabling early detection of quality issues and adjustments to production parameters to maintain product quality.
-
Supply Chain Optimization: ML algorithms can optimize supply chain logistics and inventory management in jet fuel production facilities. By analyzing historical demand patterns, market trends, and transportation constraints, ML models can forecast future demand for jet fuel and optimize inventory levels and distribution routes accordingly. ML-based demand forecasting can also help suppliers and distributors anticipate fluctuations in demand and adjust procurement and delivery schedules to minimize costs and ensure timely delivery.
-
Energy Efficiency: Jet fuel production is energy-intensive, and optimizing energy usage is crucial for reducing costs and minimizing environmental impact. ML algorithms can analyze energy consumption data and identify opportunities for energy savings through process optimization, equipment upgrades, and energy management strategies. ML-based predictive maintenance can also help identify inefficient equipment or components that may be contributing to energy waste and prioritize maintenance or replacement efforts to improve energy efficiency.
-
Environmental Monitoring and Compliance: Jet fuel production facilities must adhere to strict environmental regulations and emissions standards. ML algorithms can analyze sensor data and environmental monitoring data to detect emissions anomalies and ensure compliance with regulatory requirements. ML models can also predict the environmental impact of jet fuel production activities and recommend mitigation measures to minimize emissions and environmental footprint.
-
Risk Management: ML techniques can assess operational risks and safety hazards in jet fuel production facilities by analyzing historical incident data and identifying potential risk factors. ML-based risk assessment models can predict the likelihood and severity of accidents or incidents based on factors such as process conditions, equipment reliability, and operator behavior. By identifying high-risk areas and scenarios, ML models can help prioritize safety measures and preventive maintenance activities to mitigate risks and ensure the safety of personnel and assets.