How Can Predictive Analytics Be Used to Tackle Fraud At Policy Inception?
Below is the continuation of the transcript of a Webinar hosted by InetSoft in May 2018 on the topic of Data Analytics in the Insurance Industry. The presenter is Christopher Wren, principal at TFI Consulting. .
The next question I have is more of a business question. How can predictive analytics be used to tackle fraud at policy inception? So it's a very specific question, and maybe Christopher, if I could perhaps pass to you.
Christopher: Thanks, Jessica. This is a terrible question really because the statistics indicate that in 10 people one of those 10 will commit fraud at the point of claim, and possibly two out of the 10 will possibly agree with what they've done. That's a worrying statistic, but of course, insurance is about possibility rather than certainty. I think we get in very interesting territory around how we predict a customer's propensity to take a particular type of behavior at this early point in the process, the underwriting process.
Having said that certainly there maybe some indicators which might affect the price which we offer to a customer which might discourage them, shall we say. Ultimately of course, the customer's behavior may be based on the context of the situation. Maybe their financial need might change between the point of buying a policy and submitting the claim, for example.
Market conditions might change in the performance of the insurer at the point of claim and might influence how the customer penalizes the insurance company. For example, we know that this insurance company performs particularly badly at the point of claim. The customer may well penalize them in some way.
#1 Ranking: Read how InetSoft was rated #1 for user adoption in G2's user survey-based index |
|
Read More |
Very interesting metrics are emerging. It is important also that we take into account the policy position. I'm concern sometimes that people who focus on technology fail to recognize that there are policy issues and legal issues to think about. For example, if a customer was declined coverage on the basis of something they may or may not do, then they have an obligation to declare that insurance had been declined to all future insurers, and that takes us into very interesting legal territory.
I think finally on the question of fraud analytics, although we think about customer fraud and policy holder fraud, there are, of course, quite big issues around supplier fraud which we generally refer to as claims leakages. I think that predictive analytics has a major part to play in the way that we manage our supply chain and optimize it, and effectively insurers pay no more than the absolute requirement.
Jessica: Okay thanks, Christopher. This is another slightly more technical question coming in about the importance of real time deployment. How would the analytics guess these outputs that we're generating either around historical data or the actual data that is being given to the call center agent. How would the technology guess these outputs back to the agent in real time to make a difference at that inflection point? Natalie, would you want to take this one?
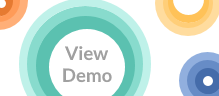 |
View a 2-minute demonstration of InetSoft's easy, agile, and robust BI software. |
Natalie: Yes, I'll take that. It's not necessarily for both, but it is very much doable, and it involved the use of APIs integrated within your system to ensure that the scoring mechanisms are relayed back to your contact management system in a real time fashion. They can be generally relatively easy to develop, and it just takes the right people and the proper building blocks to be able to get the output at one end if you like, to the presentation of those outputs and on the screen for the agent on the other end.
How Do Credit Card Issuers Use Predictive Analytics to Fight Fraud?
Credit card issuers employ predictive analytics techniques to detect and prevent fraudulent transactions effectively. These techniques leverage historical transaction data, behavioral patterns, and advanced algorithms to identify suspicious activities in real-time. Here's how credit card issuers use predictive analytics to fight fraud:
-
Anomaly Detection: Predictive analytics algorithms analyze vast amounts of transaction data to establish baseline patterns of normal behavior for individual cardholders. Any deviations from these patterns, such as unusual purchase locations, transaction amounts, or spending frequencies, are flagged as potential anomalies. Machine learning models trained on historical data can continuously learn and adapt to evolving fraud patterns, enabling early detection of fraudulent activities that deviate from normal behavior.
-
Behavioral Analysis: Predictive analytics tools analyze cardholders' spending habits, transaction history, and behavioral patterns to identify anomalies and suspicious activities. By comparing current transactions to past behavior, predictive models can detect changes in spending patterns, transaction types, or geographic locations that may indicate fraudulent behavior. Behavioral analysis techniques enable credit card issuers to detect fraud even in cases where traditional rule-based systems may fail to identify suspicious activities.
-
Transaction Monitoring: Credit card issuers use predictive analytics to monitor transactions in real-time and assess their risk levels based on various factors such as transaction amount, merchant category, location, and time of day. Predictive models assign risk scores to each transaction and flag those with high-risk attributes for further investigation or verification. By leveraging historical data and machine learning algorithms, credit card issuers can accurately identify potentially fraudulent transactions while minimizing false positives.
-
Identity Verification: Predictive analytics techniques are used to verify cardholders' identities and detect identity theft or account takeover fraud. Machine learning models analyze multiple data points, including biometric data, device fingerprints, IP addresses, and behavioral patterns, to assess the likelihood of fraudulent activity. By comparing current interactions with established user profiles, predictive models can detect inconsistencies or anomalies that may indicate unauthorized access or fraudulent behavior.
-
Fraud Detection Rules Optimization: Credit card issuers continuously refine and optimize fraud detection rules and algorithms based on feedback from historical fraud cases and emerging fraud trends. Predictive analytics tools analyze the performance of existing rules and models, identify areas for improvement, and suggest adjustments to enhance fraud detection accuracy and efficiency. By leveraging predictive analytics for rule optimization, credit card issuers can stay ahead of fraudsters and adapt to evolving fraud patterns effectively.