#1 Ranking: Read how InetSoft was rated #1 for user adoption in G2's user survey-based index |
|
Read More |
What Is Reading Comprehension for Big Data?
We all had those reading comprehension assessments back in school. But, in big data, it's no longer just about understanding the plot of a story or the main points of an article. Instead, it involves the ability to extract meaning from vast datasets, understand complex patterns, and uncover hidden insights. Remember that big data itself refers to these extremely large volumes of data that come from multiple sources and appear in diverse forms. Therefore, reading comprehension in big data requires not only the skill to process and understand written information– but also the capacity to interpret data structures, statistical analyses, and machine learning models.
Top Metrics in Big Data Reading Comprehension
Pinpointing the key metric for tracking reading comprehension can feel like searching for a needle in a haystack.
The thing is, there's no such thing as must-track metrics for everyone, as different datasets and objectives demand unique approaches. However, amidst this complexity, there are some essential, common metrics that you should consider tracking when it comes to big data reading comprehension.
F1 Score
When you want to consider both precision and recall simultaneously in your evaluation, F1 score gives equal weight to both metrics.
The F1 score can help you evaluate the overall effectiveness of your algorithm or model in extracting meaningful insights from large volumes of text data. By considering both precision and recall, the F1 score provides a more comprehensive assessment of the model's performance than either metric alone.
Four essential components:
- True Positives (TP): Number of samples correctly predicted as "positive."
- False Positives (FP): Number of samples wrongly predicted as "positive."
- True Negatives (TN): Number of samples correctly predicted as "negative."
- False Negatives (FN): Number of samples wrongly predicted as "negative."
Precision
Precision measures the accuracy of the positive predictions made by the model. It is calculated as the ratio of true positive predictions to the total number of positive predictions made by the model, regardless of whether they were correct or incorrect.
High precision indicates that the model makes fewer false positive predictions, meaning it correctly identifies relevant information without many false alarms.
Recall
Recall, also known as sensitivity, measures the model's ability to correctly identify all relevant instances in the dataset. It is calculated as the ratio of true positive predictions to the total number of actual positive instances in the dataset.
High recall indicates that the model captures a large proportion of the relevant information in the dataset.
The F1 score combines precision and recall into a single metric, offering a balanced evaluation of the model's performance. It is calculated as the harmonic mean of precision and recall:
The harmonic mean gives more weight to lower values, meaning that the F1 score will be high only if both precision and recall are high. As a result, the F1 score penalizes models that have high precision but low recall or vice versa.
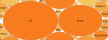 |
Read how InetSoft saves money and resources with deployment flexibility. |
Multimodal Comprehension
Multimodal comprehension refers to the ability of a system, typically an AI model, to understand and integrate information from multiple modalities, such as text, images, audio, video, or other forms of data.
In the context of big data reading comprehension, multimodal comprehension becomes particularly important because big data often comprises diverse types of information beyond just textual data.
For example, a company analyzing email outreach data as part of its big data reading comprehension task, multimodal comprehension would involve not only understanding the textual content of the emails but also integrating information from other modalities that might accompany the text.
These modalities could include:
- Images: Email communications may include images or graphics as attachments or embedded within the email body. Multimodal comprehension would require the AI model to analyze these images to extract relevant information, such as product photos, infographics, or visual data.
- Audio: Some email communications might include audio files, such as voice messages or recordings. Multimodal comprehension would involve processing these audio files to extract spoken content or other auditory cues that could provide additional context or insights.
- Metadata: Beyond the textual content of the emails, there may be metadata associated with each communication, such as sender information, timestamps, email headers, and other structured data. Multimodal comprehension would involve integrating this metadata with the textual content to provide a more comprehensive understanding of the communication patterns and context.
- Links and URLs: Emails often contain links to external resources, such as webpages, documents, or multimedia content. Multimodal comprehension would involve following these links to access and analyze the linked resources, which could include text, images, videos, or other types of data.
Human-like Understanding
Human-like understanding in the context of big data reading comprehension refers to the ability of AI systems to comprehend text and extract meaning from data in a manner that closely resembles human understanding.
This involves not only accurately processing and interpreting the information presented but also demonstrating a level of comprehension that mirrors human cognitive capabilities.
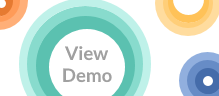 |
View a 2-minute demonstration of InetSoft's easy, agile, and robust BI software. |
Is a word cloud a way to visualize reading comprehension in Big Data?
A word cloud can indeed offer a glimpse into reading comprehension within big data, but it's not necessarily the most comprehensive or sophisticated method available. Let's delve into this. Reading comprehension involves understanding the meaning and context of the text. In big data, this could mean analyzing vast amounts of textual data, such as articles, reports, social media posts, and more. Visualizing this comprehension can be challenging due to the sheer volume of data involved.
A word cloud provides a basic visualization by displaying the most frequently occurring words in a given text, with the size of each word representing its frequency. This can give a quick snapshot of the main topics or themes present in the data. However, it lacks depth in terms of analyzing the nuances of comprehension. Here's why:
-
Limited Context: A word cloud only shows individual words and their frequency, without considering their relationship with each other or the broader context of the text. It doesn't distinguish between key concepts and less relevant terms, which can be crucial for understanding comprehension.
-
Ignores Semantic Meaning: Word clouds treat all words equally, regardless of their semantic importance. Important keywords may get overshadowed by common, less meaningful terms simply because they appear more frequently.
-
Lack of Sentiment Analysis: Reading comprehension often involves understanding not just what is said but also the sentiment behind it. A word cloud doesn't capture sentiment or tone, which can be crucial for understanding the overall message of a text.
-
No Structural Analysis: Understanding comprehension often involves analyzing the structure of the text, such as identifying main ideas, supporting details, and relationships between different parts. A word cloud flattens this structure by focusing solely on word frequency.