What Are the Costs of Purchasing and Maintaining a Data Warehouse?
Acquiring and maintaining a data warehouse involves several costs, both upfront and ongoing. It's important to consider these expenses when planning for the implementation of a data warehouse. Keep in mind that the specific costs can vary widely depending on factors like the size of the organization, the complexity of the data infrastructure, and the chosen technology stack. Here's a comprehensive breakdown of the costs associated with a data warehouse:
-
Hardware and Software Costs:
- Hardware: This includes the physical servers and storage devices needed to host the data warehouse. It can be on-premises, in the cloud, or a combination (hybrid).
- Software: This covers the licenses and subscriptions for the data warehouse software itself. Some popular data warehousing solutions include Amazon Redshift, Google BigQuery, Microsoft Azure SQL Data Warehouse, and Snowflake.
-
Data Integration and ETL (Extract, Transform, Load):
- ETL Tools: Depending on the complexity of your data sources, you may need specialized ETL tools. These tools help in extracting data from various sources, transforming it into a usable format, and then loading it into the data warehouse.
- Data Integration Services: This includes the cost of services or personnel responsible for setting up and maintaining data pipelines.
-
Implementation and Development:
- Consulting or Professional Services: Many organizations require the assistance of consultants or specialists to design and implement the data warehouse architecture, especially for complex projects.
-
Training and Education:
- Employee Training: It's essential to train the staff who will be working with the data warehouse. This includes training on ETL processes, data modeling, querying, and more.
-
Data Quality and Governance:
- Data Quality Tools: These tools help in ensuring that the data in the warehouse is accurate, consistent, and reliable. They might include data profiling, data cleansing, and data validation tools.
- Governance Framework: Establishing data governance policies and practices is crucial for maintaining data integrity.
-
Security and Compliance:
- Security Measures: This includes the cost of implementing security measures to protect the data warehouse from unauthorized access or cyber threats.
- Compliance Costs: Depending on your industry, there might be specific regulatory requirements that you need to adhere to.
-
Scaling and Upgrades:
- Scaling Costs: As your data volume grows, you may need to scale your data warehouse. This could involve upgrading hardware, increasing storage capacity, or moving to a larger cloud instance.
- Software Upgrades: Regular updates and patches for the data warehouse software.
-
Monitoring and Maintenance:
- Monitoring Tools: Tools for tracking performance, uptime, and usage of the data warehouse.
- Maintenance Costs: Routine tasks like backups, data consistency checks, and general system maintenance.
-
Licensing and Subscription Fees:
- Renewal Fees: If you're using a cloud-based data warehouse, you'll have ongoing subscription fees based on usage.
-
Opportunity Costs:
- Time and Resources: Consider the time and resources spent on data warehouse implementation and maintenance that could have been allocated to other projects.
-
Customization and Additional Features:
- Custom Development: If you require custom features or functionalities, there could be additional development costs.
What Are Some Options for Avoiding a Data Warehouse?
-
Data Lakes:
- Description: A data lake is a storage repository that can hold vast amounts of raw data in its native format. It allows for both structured and unstructured data to be stored at any scale.
- Advantages:
- Flexibility in handling diverse data types and formats.
- Cost-effective storage of large volumes of data.
- Enables data exploration and analysis without upfront structuring.
- Considerations:
- Requires strong data governance to prevent data swamp scenarios.
- Might require specialized tools for effective querying and analytics.
-
Real-time Data Processing Platforms:
- Description: Platforms like Apache Kafka, Apache Flink, and Apache Spark Streaming enable the processing of data in real-time or near-real-time, without the need for persistent storage before analysis.
- Advantages:
- Enables immediate insights for time-sensitive applications.
- Reduces the need for storing large volumes of historical data.
- Considerations:
- Requires specialized expertise in stream processing.
-
In-memory Databases:
- Description: These databases store data in RAM rather than on disk, allowing for extremely fast access to data.
- Advantages:
- High-speed data access for real-time analytics.
- Well-suited for applications that require rapid data retrieval.
- Considerations:
- Limited by the amount of available RAM, which can impact scalability.
-
NoSQL Databases:
- Description: NoSQL databases like MongoDB, Cassandra, and Couchbase are designed to handle large volumes of unstructured or semi-structured data.
- Advantages:
- Flexible schema for handling various data types.
- Scalable for high volumes of data.
- Considerations:
- Might not provide the same level of analytical capabilities as a data warehouse.
BI Platform with a Data Mashup Engine:
- Description: A Business Intelligence (BI) platform with a Data Mashup Engine is a software solution that combines the capabilities of BI tools with advanced data integration and transformation features. It allows users to blend, merge, and transform data from various sources for the purpose of analysis and reporting.
- Advantages:
- Enables users to quickly adapt to changing data requirements and integrate new data sources without heavy reliance on IT teams.
- Helps break down silos between different data sources and departments, promoting a more holistic understanding of the business.
-
Data Virtualization:
- Description: Data virtualization allows organizations to access and manipulate data without physically storing it in a centralized repository. It provides a logical view of distributed data sources.
- Advantages:
- Reduces data duplication and storage costs.
- Provides a unified view of data from multiple sources.
- Considerations:
- Performance might be impacted depending on the complexity of the virtualization.
-
Cloud-based Analytics Services:
- Description: Cloud platforms like AWS, Google Cloud, and Azure offer a range of analytics services that can bypass the need for a traditional data warehouse.
- Advantages:
- Pay-as-you-go pricing model can be cost-effective.
- Scalable and can handle large volumes of data.
- Considerations:
- May require data migration and integration efforts.
-
Federated Data Warehouse:
- Description: This approach involves keeping data in its source systems but providing a unified view through federation. Queries are distributed to the relevant source systems, and results are combined.
- Advantages:
- Minimizes data movement and storage.
- Maintains real-time access to source data.
- Considerations:
- Can be complex to set up and manage.
-
Data Fabric:
- Description: A data fabric is a comprehensive architecture that enables seamless data integration, access, and management across multiple data sources and environments.
- Advantages:
- Provides a unified data layer for data access and analytics.
- Supports data governance and security across disparate sources.
- Considerations:
- May require a significant initial investment in architecture and tools.
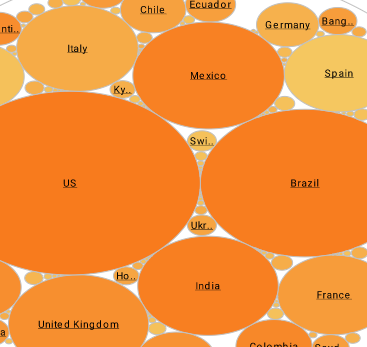 |
“Flexible product with great training and support. The product has been very useful for quickly creating dashboards and data views. Support and training has always been available to us and quick to respond.
- George R, Information Technology Specialist at Sonepar USA
|
What Is a BI Platform with a Data Mashup Engine?
A Business Intelligence (BI) platform with a Data Mashup Engine is a software solution that combines the capabilities of BI tools with advanced data integration and transformation features. It allows users to blend, merge, and transform data from various sources for the purpose of analysis and reporting. Here's a detailed breakdown of the key components:
-
Business Intelligence Platform:
- A BI platform provides tools and infrastructure for data analysis, visualization, and reporting. It enables users to create dashboards, generate reports, and gain insights from data.
-
Data Mashup Engine:
- A Data Mashup Engine is a specialized component within a BI platform that facilitates the process of combining data from multiple sources. It includes features for data integration, transformation, and blending.
-
Key Features of a Data Mashup Engine:
-
Data Integration:
- Allows for the extraction of data from various sources, which can include databases, spreadsheets, web services, and other structured and semi-structured data formats.
-
Data Transformation:
- Enables users to clean, filter, aggregate, and manipulate data before it is used for analysis. This ensures that data is in a suitable format for meaningful insights.
-
Data Blending:
- Combines data from different sources into a single dataset, allowing for more comprehensive and holistic analysis.
-
Data Enrichment:
- Enhances raw data by adding supplementary information or calculated metrics to provide a more comprehensive view.
-
Data Quality and Governance:
- Provides tools and features to ensure data accuracy, consistency, and compliance with organizational standards.
-
Data Cataloging and Metadata Management:
- Helps users identify and understand the various datasets available, including information about their structure, source, and lineage.
-
Data Versioning and Lineage Tracking:
- Allows users to trace the history of data changes, which is crucial for auditing and ensuring data integrity.
-
Data Connectivity:
- Supports a wide range of data connectors and protocols to access data from diverse sources, including databases, cloud services, APIs, and more.
-
Benefits of a BI Platform with Data Mashup Engine:
Examples of BI platforms with robust Data Mashup Engines include tools like InetSoft, Tableau, Microsoft Power BI, QlikView, and Alteryx. These platforms empower users to extract, transform, and integrate data from diverse sources, resulting in more meaningful and actionable insights.
More Articles Related to Data Warehousing Alternatives
Bring in the Other Atomic Data Sources - Sure, that's an option. But you can also even bring in the other atomic data sources whatever they may be. A lot of people with service-oriented architectures have services providing various data feeds. Of course you have transactional operational databases and of course data marts, warehouses, and the like. Even external feeds from vendors can become one of...
Business Intelligence and Data Warehousing - InetSoft's business intelligence solution for data warehousing environments allows enterprises to mashup multiple data sources to offer interactive dashboards and production reporting. The software can access multiple, disparate databases, via any JDBC connector as well as OLAP cubes, ERP applications, spreadsheets, flat files, Web services and other databases. The Web-based interface provides an...
Consistent Customer Data Across The Enterprise - That is also being called conforming dimensions for those that have been involved in data warehousing and data modeling. CDI, or Customer Data Integration, is consistent customer data across the enterprise. Again, this is a not new concept, but it is a new name, CDI. Remember 360 degrees around the customer, customer relationship management...
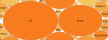 |
Read how InetSoft saves money and resources with deployment flexibility. |
Dashboard Software for Accessing SQL Databases and Data Warehouses - Looking for software for accessing SQL databases and data warehouses? InetSoft offers Web-based BI software that can mashup data from these and other sources for dashboard reporting. Let's talk about this enterprise implication. Large organizations have lots of rules and regulations, and there are reasons for all that stuff. We hear all about data governance these days and obviously it's a big deal, especially for public companies because they...
Data Mashup Has To Be Tightly Integrated - It's also not really that viable as a plug-in to a tool because with that level of self-service that you are trying to provide, it really has to be tightly integrated into the rest of the user experience. For maximum ease of use, you wouldn't want a separate tool with a separate interface providing the mashup and then having clunkiness around trying to get that resulting data to be a source for the reports or dashboards or scorecards, etc...
Definition of an OLAP Cube - An OLAP cube is a specific method of storing massive amounts of data in a data warehousing environment. The term "cube" refers to the numerous defined dimensions, measures, and hierarchies by which users designate their data to be stored and constructed for in-depth analysis...
Do You Assume You Need ETL Tools? - Do you assume you need ETL tools and a data warehouse for a BI solution? Our data dashboard tools offer Web based reporting and dashboard software that accesses disparate data sources directly. If you already have an ETL process established and running smoothly, InetSoft's Style Intelligence...
Does Virtualized Data Access Mean The End Of Data Warehousing? - One has to remember that the enterprise data warehouse and the architecture around it is very much a child of the 1980s, the technology of the '80s, the organization of the '80s and indeed, the business drivers of the '80s, and that doesn't mean it's wrong. A lot of those things are still existing, and they are still part of the culture...
Evaluate InetSoft's Transaction Reporting Software - Looking for good transaction reporting software? InetSoft's pioneering dashboard reporting application produces reports and analytics for any transaction system. View a demo and try interactive examples...
Information About Visualization Products - This is a table of contents of useful product information about, and benefits of, InetSoft's visualization capabilities built into its business intelligence software for dashboards, reporting, and analytics. Now let's talk about advanced visualization. This one is really about time to insight. Or showing us what is beneath the tip of the iceberg. At the tip of the iceberg, that's where the BI industry does really well...
Pervasive BI Architecture Includes Transactional Services - The pervasive BI architecture includes transactional services, for example, OLTP, that provide enterprise bookkeeping functions. This is where we find traditional call center automation, operational CRM, enterprise resource planning, supply chain management, and legacy applications. Data integration services bridge multiple domains providing...
Problems of Inefficient Business Intelligence - I am sure that there are a lot of you who have gone through this process. The main problems are self-evident. The process is predominantly made up of manual processes. Even in the scenario I gave for automating the actual budget capture or data entry through a spreadsheet, there is the manual process of distribution and compiling and tracking and...
Solution for Self-hosted Business Intelligence - Are you looking for a good self hosted business intelligence? Unlike many other modern BI providers, InetSoft's server-based solution is designed to be hosted wherever you want it. You are free to choose whichever cloud computing service or install the application in your own network. Self-hosted BI solutions can offer better performance and faster data processing since they are deployed within the organization's own network...
Superstore Analytic Dashboard Example - The dashboard provides a variety of filters to drill down and up for different granularity. The users can also filter the visualizations in the dashboard by region, state, category of products, sub-categories, and customer segments. It is also possible to filter the dashboard with 3 sliders which can determine the total amount of sales, and the amount of profit and one slider to...
Tool For Data Visualization Storytelling - What do business decision makers look for when choosing the best data visualization storytelling tool for their company? Factors to consider include the ability to mashup different types of data sources, the ability to import data, integration with data warehousing, options for prototyping new data, accessibility, and user-friendly features that speed up decision making...
Web Based Interactive Dashboard Via HTML5 - Looking for interactive dashboards via Flash or HTML5? Since 1996 InetSoft has been making business dashboard software that is easy to deploy and use. Now that Flash has been sunsetted, InetSoft's Web-based server solution delivers fully interactive dashboards in HTML5. With cutting-edge data mashup technology, data visualization tools, and a flexible product...
What Are Data Warehouse BI Tools? - They are tools that manage, manipulate, transform, query, or visualize data that is stored in OLAP databases. Originally a user had to be a BI expert to use such tools, but nowadays even business users can use interactive dashboards to answer questions from the data warehouse. Just like InetSoft, Power BI is not a data warehouse tool. It is a BI tool that can be used with a data...