From an efficiency perspective, data mashup solutions offer a significant cost reduction compared to replication based strategies, but even more importantly from an ongoing standpoint, for future BI solutions and reacting the changes, development time is significantly shorter and therefore cheaper. Typical projects take four to six weeks in any of those examples that I have shown you here.
ROI for initial projects can be less than six weeks, and you are always building this data services capability as you go. InetSoft is very well recognized in this industry as a leader in data mashup, and our one BI product has the broadest range of capabilities and flexibility and yields very high performance.
Let’s turn to the audience questions. We have two questions that are very similar that we can address at the same time. One of them is on the limits of data virtualization in terms of dataset size and performance? And the other one is a question on performance assessment.
#1 Ranking: Read how InetSoft was rated #1 for user adoption in G2's user survey-based index |
|
Read More |
What Environments Does Data Virtualization Handle?
As we have mentioned, it depends on the expectations of the business. Data virtualization handles mainly two types environments. The first is operational environments without many data sources but large numbers of users, a 100, 1,000 or 10,000, where low latencies are required. This is typical of call enter, and the application is so see the entire customer view. Here you have got no baseline on data virtualization tools capability of matching congruent connections and optimizing combinations from those data sources.
The other type of environment is the business intelligence environment. The traditional BI environment typically required access to a lower number of data sources, but nowadays they require access to many data sources. The requirements are for simple agile reports in BI solutions and mission-critical reports. The answer to performance questions in both environments is a matter of scaling up with off-the shelf-servers and setting up data grid caches. Our BI solution supports load balancing and clustering, so relatively economically, you can scale up to provide high performance in high-user, large data volume scenarios.
Another question here is about how most ERP and CRM vendors still are designed to work only with their own resident data. Can this architecture be tricked to use virtualized data as if it is its own data?
Yes, the data is local, I think this is an excellent question. The problem is these vendors don’t often up their UI to visualize the additional data you may want to virtualize. So you have to go the other direction and use our BI application to visualize the virtualized data, which would include the ERP or CRM data since we have connectors to most of them.
One other question here, big data is becoming an important buzzword and many companies are seeing the reality of this. What's the role of data virtualization with management of big data? Is this a database replacement for big data frameworks such as Hadoop, Apache, etc?
Data virtualization plays a key role in big data implementations, but honestly it’s not exactly a replacement. Big data frameworks such as BigTable, Hadoop, or parallel databases or columnar databases, column based databases are very specialized tools in data management processes. They do a great job there. But it takes a lot of time to process the BI needs associated with the data. Our application is the better BI front-end to this data rather than what comes with those solutions.
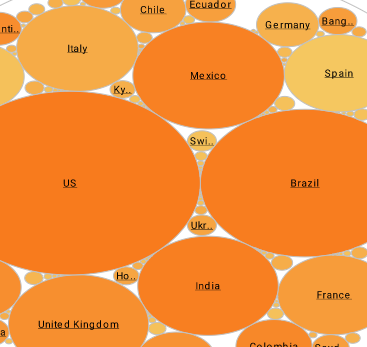 |
“Flexible product with great training and support. The product has been very useful for quickly creating dashboards and data views. Support and training has always been available to us and quick to respond.
- George R, Information Technology Specialist at Sonepar USA
|
What kinds of ROI can be expected with a data mashup project?
It really depends on the kind of project where data mashup or data virtualization is being implemented. Typically if you are talking about it at a project level, and I am going to take the example of a BI or a reporting project. The ROI is very much based on the time that it would take for you to take a more waterfall approach to the BI or reporting project or customer intelligence project where you are gathering a lot of the requirements, getting the data model for it exactly right for creating this virtualization or data model. Then you are start your ETL programmers working to extract all the information. Then you start the reporting development.
With data virtualization it’s an interactive process. It’s agile BI development. You start building the data model, then the dashboards or reports. In a couple of weeks your business partner looks at them and says ‘no, I want that calculation added and that KPI added and more information added and you evolve to the right solution much faster and in the process you have also not created replicated data stores, bought additional hardware or software storage to support all of that.
Right there is where you can get a six week ROI. But if you start now thinking about how this can be evolved and changed it can be much higher and that's not really even counting the value you get from those reports. When I switch over to customer information kinds of information, there the value is not so much the cost. It's really the value of how much new insights are you bringing into the customer interaction process and what's the value of that to you in terms of incremental revenue or retained revenue or increased lifetime.
More Articles About Data Mashup
Big Data Job Turned into a Small Effort - "I took on a job that involved analyzing a large amount of complex data. I was really worried that I had bitten off more then I could chew. When I found InetSoft's Style Intelligence I knew I would be fine. The multi-dimensional charting combined with the ability to really drill down makes this platform one-of-a-kind. They also gave me plenty of options for showing off the results for my finished project...
Fuel Consumption Rate - The fuel consumption rate is one of the main KPIs tracked on fuel management system dashboards. This statistic assists companies in monitoring the fuel consumption of their equipment or vehicles over a certain time frame. Businesses may make educated judgments to optimize fuel use by identifying patterns, trends, and anomalies in fuel usage by studying this data. Keeping...
Meteorological Parameters - Apart from particular contaminants, meteorological indicators are also included as critical KPIs in air quality dashboards. These consist of wind direction, speed, temperature, and humidity. Since climatic conditions affect how pollutants disperse and change, understanding them is essential for interpreting data on air quality. For instance, the direction and speed of the wind influence...
Policy Administration Key Metrics - Policy Issuance Time: This statistic tracks how long it takes to process an application and issue a policy. To guarantee prompt policy issuance and enhance the client experience, insurance operations analysts track this KPI. Policy Renewal Rate: Analysts can gauge client loyalty and pinpoint opportunities for development...