This is the heat map view of performance. So in this chart, what I’ve colored in are the funds that are selected. Let’s bring everything back for a second. What the heat map is doing is taking all 1,800 funds and grouping them into the categories: growth international, balanced, small cap, and so forth.
So this top left box is the Fidelity Magellan Fund. It is old data, but it’s stating the three year performance is 22%. What is good is green. The scale goes from 43 as dark green to minus 26 at bright red. The box is big because of big invested position. This gives me at-a-glance views of what is happening in this portfolio.
I see the growth category is generally doing fine. The international funds are a different story. It has got one that’s doing really well, a small one. One is doing bad. In general it’s a lighter color so it’s doing less well. Whereas the treasury category, it’s a more middle of the road performance, but very consistent. The capital appreciation category has a couple of extreme outliers.
Let’s go back to our selection. This is that top right quadrant again. This is another way of quickly getting across where my concentration is in my portfolio. It’s heavily skewed towards three fundcategories, the growth, small cap, and capital appreciation. There is not much anywhere else especially when you look at the invested positions.
#1 Ranking: Read how InetSoft was rated #1 for user adoption in G2's user survey-based index |
|
Read More |
So I’m finishing up. I might want to take this view, and say I really like this, I want to give it to my team so I go to the task view, and export the view. We saw how to export data a few minutes ago. Let’s export the chart. Send this to a PowerPoint, and put on my desktop. So now I’ve got the chart out of Style Intelligence in the PowerPoint. These are all editable. They’re just PowerPoint objects, so I can take it to my team and say here is my top right quadrant and is what I’m excited about, and here is I can see the concentration right there.
Now let’s turn back to the visual analysis. I’ve shown pretty much as much as I can do visually. Let’s run a predictive model now to go deeper in the analysis. I go to the task view. Build a predictive model, and this is something really unique about an integrated in-memory, visualization modeling tool that has all the data. I know what my selection state is so I create the model. I called it Top Right Quadrant.
I’ve got only one table in this. It’s a simple data set. I can do a regression model against any of the numeric fields in this table that are loaded in, or I could do a classification model. I want to do one of those classification models that is going to take this group of selected funds into our regression against the entire population, which is everything else.
I need to analyze performance and risk. So I want the best model. About 25 models run behind the scenes, and it’s picking the ones that’s’ the best fit. So it’s a very rich set of regressions that I just ran, and it checks that I have enough fields to be explanatory and that I have enough rows to be predicted if I want to score others for their potential to be in this quadrant.
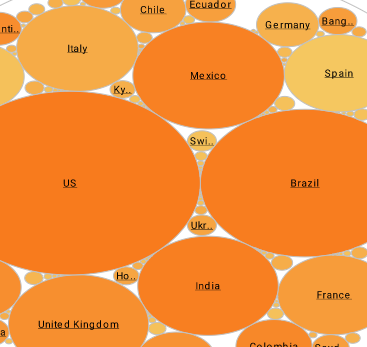 |
“Flexible product with great training and support. The product has been very useful for quickly creating dashboards and data views. Support and training has always been available to us and quick to respond.
- George R, Information Technology Specialist at Sonepar USA
|
It tells me that fund category explains 52% of performance, so what we saw visually we see here the one that’s the highest return is small cap. It’s basically figured out this correlation, and the next one is the capital appreciation, and the next strongest factor is the affiliation which is this one.
There are some other fields. For example, look down the list here. Return since inception. That’s another return rate. Let’s edit that out. We just saw we made a mistake when we ran the model. It’s very easy to do this. I just uncheck it. It’s no longer explanatory field. I rebuild the model so that way it’s going to be taken off that list. So now I can scan the other ones here and get the feel for what makes up this performance.
But this is an example of a great way to do visual discovery and then combine it with quantitative modeling to get a feel for the overall makeup of a portfolio and now again it can predict, and it will run the model against the rest of the field to put two more fields in the table and put a field for predicted in a score. Then I could go and look at which of these other fund had the same characteristic. It would be predicted to have the same behavior. Obviously this kind of capability can be used in a whole wide range of areas from impact on sales results, to impact on giving, to impacts that breakdown in a factory floor process on yields. When we use it, the mind can really probably envision a lot of things here.