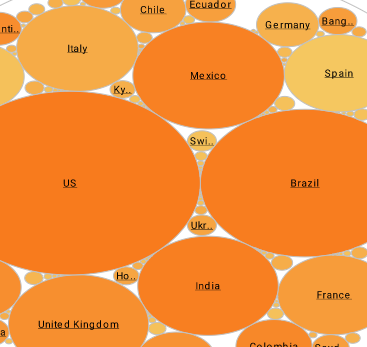 |
“Flexible product with great training and support. The product has been very useful for quickly creating dashboards and data views. Support and training has always been available to us and quick to respond.
- George R, Information Technology Specialist at Sonepar USA
|
I think there is also a general distinguishing feature between analytical and operational MDM, and that is one that we see as well where analytical is more about supporting BI and reporting and analysis activities, including things like reporting hierarchies, whereas operational MDM is more about establishing a gold copy or reference data that can be used by multiple transaction-oriented applications.
So that’s one distinction that people should look at when they are looking at tools and looking at technology to combat the problem.
And then you do have both small vendors and large talking about MDM, and of course, as you get to the larger vendors, it often includes a lot of services approaches that go with the software. So again, organizations have to look at what they really want to take on in terms of build versus buy and bringing in tools themselves that their IT staff can work with versus outsourcing a lot of the effort to more service-oriented organizations.
I think the first step is to see what assets and processes are already in place to handle some of the key activities involved in MDM such as data integration and the profiling and discovery and business rules management that will be involved. Then you can see what’s missing from your current set of tools and current set of technology expertise in the organization to accomplish a specific business purpose identified.
Another key here I think is to make MDM efforts repeatable so you don’t want to have to reinvent the wheel as you go from business function to business function to address MDM problems, and that’s where I get to the idea that you really need that enterprise view ultimately with MDM.
So that way as you are consolidating systems throughout the organization, particularly after mergers and acquisitions, you are able to move from one scenario to another and set up rules and processes and workflow that will be working again and again and can be refined and continuously improved as you go along.
Then a final recommendation, I think IT and the business side need to be in agreement over goals, no question about that, so that both can be happy with the deliverables and also that filters into how the technology acquisition works and whether those are successful.
Since master data management supports all different kinds of departments and initiatives, how does funding for master data management work? Who pays for it, and how should it work?
That's an age-old issue, certainly for IT. How to get business departments and business functions to pay for things that don’t seem to elicit an obvious payback? So I think that’s where it's important again for IT and business to begin to work together to establish specific deliverables and solve specific business problems. So that’s a good way to get funding because I think if the business side can see how we are going to solve a customer data problem that will lead to more use of customer self-service which is going to drive down their cost, then that’s obviously something they are going to be very interested in.
I think going back again a little bit to the analytical and operational MDM differences, I think there you can see that certainly on the analytical side you are going to have finance very interested in this because it's going to improve their reporting hierarchies and make them more agile that way.
When hierarchies are changed or altered, particularly again through acquisition or re-shifting of sales territories in other departments, it isn’t going to involve an enormous effort to redo the entire system of reporting in an organization. So MDM can be very useful and drive down cost there.
On the operations side, every organization is trying to get their applications integrated. That’s been going on for a long time, and the information integration side of it is some of the most difficult to do. And so MDM is being applied there within certain ERP systems, but obviously most organizations have multiple systems.
They might have, for example, Oracle and SAP, or even if they have all the different systems that say Oracle has been acquiring lately, these systems are still discreet enough that MDM is something that they really need to be looking at in terms of unifying the applications and improving the information flow and therefore getting more return on investment from those applications. So there I think again, an MDM effort can attract interest from supply chain, manufacturing, inventory management people if, again, it can be shown there is a direct business benefit to what they are trying to do.
How should MDM success be measured? How do you know you have done it successfully?
Well, again, and I keep repeating myself, but I think that if you look at it again through business benefits, that’s how it really should be measured. I think that's what's distinct about MDM from previous integration efforts is that if you can point to a business problem that was improved or resolved, cost being driven down, not only in IT, but that’s certainly a significant issue IT cost, but also on the business side, as well. That’s really success in terms of MDM.
Because it’s little bit difficult to measure in terms of amount of data reconciled or number of data quality problems solved, just on that basis alone, because I think organizations are going to have data quality and data inconsistency issues they are going to have to resolve. They are never going to get to the end of it. So it's better to just look at the business issues that are being resolved. I think it’s another big issue with MDM to be able to set in place the rules and the workflow and the processes so that you can move from one issue to the next. In the end you will start to build this horizontal function that will begin to address a much greater number of problems that the organization is facing in terms of data inconsistency and information quality problems.
So I think there are three things really, to be incremental, to address business problems and measure the success in terms of business problems solved and then establish the process and the workflow ability so that the solution can be repeatable.
What Are Some the Problems That Can Arise When There Is Poor Master Data Management?
Poor master data management (MDM) can lead to a myriad of problems across various aspects of an organization's operations, impacting efficiency, decision-making, compliance, and customer satisfaction. Master data refers to the core data entities that are essential to the operations of a business, such as customer data, product data, employee data, and supplier data. Effective management of this master data is crucial for ensuring data accuracy, consistency, and reliability. When MDM practices are lacking or inadequate, several problems can arise:
-
Data Inaccuracy and Inconsistency: Without proper MDM processes in place, master data can become inaccurate, incomplete, or inconsistent. This can occur due to data entry errors, duplicate records, or outdated information. Inaccurate and inconsistent data can lead to confusion, mistrust, and inefficiencies across the organization, as different departments may rely on conflicting data for decision-making.
-
Poor Decision-Making: Inaccurate master data can significantly impair decision-making processes at all levels of the organization. Leaders and managers rely on accurate data to make informed decisions regarding strategic planning, resource allocation, product development, and customer relationship management. When master data is unreliable, decision-makers may base their judgments on flawed or outdated information, leading to suboptimal outcomes and missed opportunities.
-
Operational Inefficiencies: Poor MDM can result in operational inefficiencies throughout the organization. For example, employees may waste time searching for the correct version of a customer record or product information, leading to delays in processing orders or resolving customer inquiries. Inconsistencies in supplier data may lead to procurement delays or errors in inventory management. These inefficiencies can increase costs, decrease productivity, and harm the organization's competitiveness.
-
Compliance Risks: Many industries are subject to regulatory requirements regarding data management and privacy, such as the General Data Protection Regulation (GDPR) in the European Union or the Health Insurance Portability and Accountability Act (HIPAA) in the United States. Poor MDM practices can expose organizations to compliance risks, as inaccurate or incomplete master data may lead to violations of data protection laws or regulatory requirements. Non-compliance can result in financial penalties, legal liabilities, and damage to the organization's reputation.
-
Customer Dissatisfaction: Inaccurate or inconsistent customer data can have detrimental effects on customer satisfaction and retention. For example, sending marketing communications to the wrong address or addressing customers by the wrong name can create a negative impression and erode trust. Inaccurate product information or pricing discrepancies can lead to customer complaints and lost sales opportunities. Poor MDM can damage the organization's reputation and brand image, ultimately driving customers away to competitors.
-
Difficulty in System Integration: Many organizations rely on multiple systems and applications to manage different aspects of their operations, such as customer relationship management (CRM) systems, enterprise resource planning (ERP) systems, and supply chain management (SCM) systems. Effective MDM is essential for ensuring seamless integration and interoperability between these systems. Poor MDM practices can create compatibility issues, data conflicts, and synchronization errors, hindering the organization's ability to leverage technology effectively and achieve its business objectives.
-
Lack of Data Governance: Data governance refers to the framework of policies, processes, and responsibilities for managing and protecting data assets within an organization. Poor MDM often goes hand in hand with a lack of robust data governance practices. Without clear roles, responsibilities, and accountability mechanisms in place, master data may be neglected or mismanaged, and undermining trust in the organization's data assets.