Mark Flaherty (MF): I absolutely believe that’s the trend. Business
users don’t want to have to go back to IT every time they want a new piece of data
or customize a dashboard. They want to see their dashboard in particular way. They want to
be empowered so that they can personalize their dashboards to their particular needs. The
benefit of data mashup is that shortens the time that it takes to get a dashboard that is
useful for a business user. They can more easily build their own.
How do you actually enable or power a user to leverage data mashups?
It has to start with the business analyst. They have to build up all the KPIs that the
organization has available. And use our software to see all the available KPIs. And then
it’s a matter of dragging and dropping them onto their dashboard canvas, and
that’s how they’re able to create their own dashboard. This is going to be a
really powerful way to let people look at data, because you don’t want to go back to
IT all the time.
#1 Ranking: Read how InetSoft was rated #1 for user adoption in G2's
user survey-based index
|
|
Read More
|
Is transparency of decision making a critical component of dashboards?
It is. Because you can, as a senior manager, see by virtue of looking down a layer of
dashboards, what people are doing, what decisions they have been making and when. That
gives you a lot of ability to understand what is happening.
Indeed, a lot of things have changed in the past ten years in the whole BI and dashboard
space. One of the biggest things we are seeing and are very excited about, because it
aligns well with our strategy, is the idea is that the KPIs and business metrics can
really escape the upper echelon of the organization and be applicable to the entire
hierarchy of an organization. And that is where we do see a tremendous amount of benefit,
because we have always found the information has been there, and definitely more so than
in the past few years. The world is more instrumented. The modern enterprise is more
instrumented. We are also seeing the trends such as SaaS and Web 2.0.
But while the information may be captured somewhere, the problem is that it’s not
always visible to everybody. And a lot of time we’ve seen organizations where in the
past they have had a BI analyst in Germany, a BI analyst in France, a BI analyst in the
UK, all responsible for generating reports. That’s where we started. And I think
today’s culture is much more one where the reliance on the BI analyst isn’t as
great any more, because information can flow in a more near real time scenario. Especially if you keep information simple, contextualized, so you know what your goals are,
what the benchmarks are. And then that information can get out to all the folks in the
organization, relevant to what their job is. I think that is where there is a huge
opportunity for performance gains.
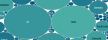 |
Read why choosing InetSoft's cloud-flexible BI provides advantages over other BI options. |
What Are the Advantages of Data Mashup vs. Data Warehouses?
Data mashups and data warehouses are two approaches to managing and utilizing data within an organization, each with its own set of advantages and use cases. Below, I'll outline the advantages of data mashups compared to data warehouses, highlighting how they differ and the benefits they offer in different scenarios.
1. Flexibility and Agility
Data Mashup:
- Dynamic Data Integration: Data mashups allow for the quick and dynamic integration of data from multiple sources without the need for pre-defined schemas or extensive data modeling. This flexibility enables organizations to respond rapidly to changing business needs, as new data sources can be incorporated on the fly.
- Ad-Hoc Analysis: Users can easily create ad-hoc reports and dashboards by combining various data sets. This is particularly beneficial in fast-paced environments where decision-makers need to access and analyze information quickly without waiting for the data to be processed through traditional ETL (Extract, Transform, Load) processes.
Data Warehouse:
- Rigid Structure: Data warehouses are typically more rigid, with a predefined schema that requires careful planning and design. While this structure ensures data consistency and integrity, it can limit the ability to adapt quickly to new data sources or changing business requirements.
2. Speed of Implementation
Data Mashup:
- Rapid Deployment: Data mashups can be set up and deployed relatively quickly since they do not require the extensive planning, design, and ETL processes that a data warehouse requires. This makes data mashups ideal for projects where time is a critical factor.
- Immediate Insights: Users can start analyzing data almost immediately after it is ingested, providing faster insights and enabling quicker decision-making.
Data Warehouse:
- Longer Implementation Time: Building a data warehouse is a more time-consuming process due to the need for data modeling, ETL pipeline development, and careful data integration planning. This makes it less suitable for situations where rapid deployment is required.
3. Cost Efficiency
Data Mashup:
- Lower Initial Costs: Data mashups typically require less upfront investment compared to data warehouses. There is no need for extensive hardware or complex ETL processes, which can reduce the initial setup costs.
- Reduced Maintenance: Since data mashups do not involve the complex structures and processes of data warehouses, they generally require less ongoing maintenance, further reducing costs.
Data Warehouse:
- Higher Initial Investment: Setting up a data warehouse often involves significant costs, including the purchase of hardware, software, and the time required for design and implementation. The ongoing maintenance of the ETL processes and data models can also add to the cost.
4. Real-Time Data Access
Data Mashup:
- Real-Time or Near Real-Time Data Integration: Data mashups can pull in data from live sources, allowing for real-time or near real-time analysis. This is particularly useful for applications where timely insights are crucial, such as monitoring social media trends, analyzing real-time sensor data, or tracking financial transactions.
- No Data Latency: Since data mashups do not rely on batch processing like traditional data warehouses, there is less latency in accessing and analyzing data, making it suitable for real-time decision-making.
Data Warehouse:
- Batch Processing: Data warehouses typically operate on batch processing, where data is collected, processed, and loaded at regular intervals (e.g., daily or weekly). While this is sufficient for many business intelligence applications, it may not meet the needs of scenarios requiring real-time data.
5. Ease of Use
Data Mashup:
- User-Friendly Tools: Many data mashup tools are designed to be user-friendly, enabling non-technical users to combine, analyze, and visualize data without requiring deep technical knowledge. This democratization of data access empowers business users to derive insights independently of IT or data teams.
- Minimal Data Transformation Requirements: Since data mashups can work with data in its original format, there is often less need for complex data transformations, making it easier for users to access and work with the data.
Data Warehouse:
- Requires Technical Expertise: Working with a data warehouse often requires a higher level of technical expertise, particularly in understanding the underlying data model and writing complex SQL queries. This can limit access to insights to those with the requisite technical skills.
6. Data Source Diversity
Data Mashup:
- Integration of Diverse Data Sources: Data mashups excel at combining data from a wide variety of sources, including unstructured data (e.g., text files, social media feeds), semi-structured data (e.g., JSON, XML), and structured data (e.g., databases, spreadsheets). This capability allows organizations to leverage a broader range of data in their analysis.
- Support for Cloud and API Data: Many modern data mashup tools are designed to integrate with cloud services and APIs, making it easier to incorporate data from SaaS applications, web services, and other modern data sources.
Data Warehouse:
- Primarily Structured Data: Data warehouses are traditionally designed to store and manage structured data, such as data from transactional systems and databases. While they can handle semi-structured data with some modifications, they are generally less flexible in integrating unstructured or diverse data sources compared to data mashups.
7. Customization and Personalization
Data Mashup:
- Highly Customizable: Data mashups allow users to create customized views of data that meet their specific needs. Whether it's a marketing team combining customer data with social media analytics or an operations team merging supply chain data with weather forecasts, the ability to tailor data mashups to specific use cases is a significant advantage.
- Personalized Dashboards: Users can easily create and modify dashboards to visualize the data in ways that are most meaningful to them, enhancing the relevance and impact of the insights.
Data Warehouse:
- Standardized Reporting: While data warehouses provide a consistent and reliable foundation for reporting, they are generally less flexible when it comes to customization. Changes to the data model or ETL processes can be time-consuming and may require significant effort.
8. Scalability and Performance
Data Mashup:
- Scalable for Ad-Hoc Use Cases: Data mashups are highly scalable for ad-hoc analysis and specific, targeted use cases. They are ideal for scenarios where the volume of data is not extreme or where the analysis needs are specific and do not require the extensive infrastructure of a data warehouse.
- On-Demand Processing: Many data mashup tools operate in the cloud, allowing organizations to scale processing power as needed, which can be more cost-effective for intermittent or seasonal workloads.
Data Warehouse:
- Optimized for Large-Scale Data: Data warehouses are designed to handle large volumes of data and complex queries efficiently, making them suitable for organizations with significant data processing and storage needs. They are ideal for enterprises that require consistent, high-performance analytics on large datasets.