And as a result we are seeing that there's a lot of demand for analytics tools and specifically features within platforms that allow users to seamlessly connect to and combine a wide variety of IOT data sources, both in the cloud and on premise. So yes, I would say the trend is how does the IOT better enable people to see and understand their own data, and I would say there's been a lot of traction, but there's still some way to go.
Abhishek: Holly, I agree with your thoughts on this. I know you, yourself, are a big user of IOT technologies to perfect your meat grilling and cooking times with the applicability both in your home life and with what we see with your customers.
Holly: Yes, I did connect my bake oven to this and that. So it's again more data. Bring it on, and it's really a huge opportunity. The difference about a lot of this data from the traditional data is, its new data. I am mixing interesting new data from internet of things.
#1 Ranking: Read how InetSoft was rated #1 for user adoption in G2's user survey-based index |
|
Read More |
New Digital User Cases Drive Analytics Demand
Although with these new digital user cases, the imperative is to analyze it because it's new. It's interesting. There's a lot of value in it, and our customers from all industries are actually interested. Many industries that you might not think of as IOT industries like high-tech industries are actually benefiting from connecting their devices to the back to their data platforms and starting to analyze that data. Bring it on. It's great.
Abhishek: And we are getting into our final three trends, and I see some great questions coming up in the queue so please keep up that up, and we will have some time at the end for them. Trend number: 8 self-service data prep becomes main stream as end users begin to shape data. Holly, can you tell us kind of what you are thinking with this one and how you see that playing up?
Holly: So this is both the opportunity and the challenge, because as we see in the increase in the variety of the data and the amount of data and the new use cases that are coming out, it's the business that knows their question, and it's the business that knows the next question and the data questions and how to find the data questions. So we have to put the data and the analysis in the hands of the business, and I think we all understand that what the implication is.
If we go back to our concept of the data like being the foundation, we have to get the data from the source to the business in a much more agile fashion, and so the self service plays a role in that. There was a question earlier on what was the implication of all this self service data? Is it only the business users who are doing to do the data preparation?
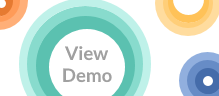 |
View a 2-minute demonstration of InetSoft's easy, agile, and robust BI software. |
Empower Business Users with Analytics
No, that's obviously not the case, but more and more business users will be empowered, and they are all being empowered to prepare or participate in the transformation of the data from source to analysis to insight, and that's a big trend, and we will see more of that happening. We will also see the balance between end user data and self service and end user data preparation and system happening. The traditional data preparation tools are coming into the balance. It's not going to be one-size-fits-all like everything else, but we will see a lot more of this happening.
And the role, if you like, or the opportunity of IT organizations is to enable that in a global manner in a way that's both high-performance and functional, but that also lowers the risk. Keeping risk lower and enabling that, we are seeing definitely more of that on the self-service side. And again the more traditional data preparation tools still have a role to play. So there is a balance there, too.