Mark Flaherty: Now let’s talk about in-memory analytics. Clearly the benefit is speed-of-thought queries. So accessing data in memory is a million times faster than accessing it via disk, and if you minimize the cost of ownership because IT has another way of speeding up queries rather than the expensive process of creating aggregates and summarizations and figuring out what to index. But the next big benefit is for answering complex business questions.
So if any of you have tried to author a challenging query, such as what are my sales versus a percent of total or who is buying both products A and B, you know that potentially involves authoring a subquery which can be really slow in a traditional relational environment where the data is coming from disk.
There is a lot of confusion in the marketplace because people will use in-memory as if it’s synonymous with advanced visualization, and it’s not. In-memory is a type of technology that different vendors, it could be advanced visualization ones, it could be a BI platform, it’s a type of technology that anyone can be taking advantage of.
#1 Ranking: Read how InetSoft was rated #1 for user adoption in G2's user survey-based index |
|
Read More |
So if we look at the current approaches to fast BI, if you have a slow query environment, usually the burden is on IT to speed up the performance, or the user might change their approach, and just because their queries time out or they take too long, they’ll ask smaller simpler questions. This is one reason why sometimes BI is left to the experts because as you move to those outer spectrums of users, they don’t have time to wait for answers to their questions.
So if you look at how traditional queries work. Somebody runs a query. The results are usually retrieved a real atonal database from disk. Then you look at those results, and you have to first say “is it what I expected to get back?” And maybe it’s not right. Maybe you forgot to add a particular filter, so you repeat this process. Depending on your environment, any single query can take a few seconds, a few minutes, or potentially hours. So the whole idea of getting to interactivity is a final step after what has been a very slow process.
Whereas with in-memory architecture, instead what happens is somebody takes a big extract, it could be from a data warehouse, it could also be from a source system, and that data is loaded into memory. From a user perspective, the user only interacts with a cached result set. So this is where many advanced visualization tools will take that approach where you are looking at a finished dashboard and interacting there. Some BI vendors, like InetSoft, will also use in-memory to accelerate even the traditional queries as part of the total BI platform. So the iterative process is fast.
When it comes to evaluating in-memory technologies, whether the software is 64-bit or 32-bit dictates how much of the memory can be addressed. 64-bit operating systems can address up to a full terabyte of data. So you can almost store your whole data mart or even your data warehouse in memory. Whereas 32-bit operating systems can only address 3 gigabytes of memory.
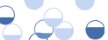 |
Read what InetSoft customers and partners have said about their selection of Style Scope for their solution for dashboard reporting. |
This innovation from an industry perspective is immature. There is no consistent approach, and it’s not widely adopted, yet, because not many customers have wide adoption of 64-bit. So it’s a good idea to plan for 64-bit down the road. This will be something that will be helpful for higher value and lower TCO and it’s something that benefits all users.
What Kinds of Analytics Are Done in Silviculture?
Silviculture, the practice of managing and cultivating forests to meet specific objectives, relies heavily on data and analytics to make informed decisions about forest health, sustainability, and productivity. Analytics in silviculture encompass a wide range of areas, from tracking forest growth to assessing environmental impacts. Here are the key types of analytics conducted in silviculture:
1. Growth and Yield Analytics
Objective: Predict forest growth and timber yield over time to optimize harvest cycles and maintain forest sustainability.
-
Forest Inventory Data: Regular data collection on tree species, age, diameter, height, and health to track forest composition and structure.
-
Growth Models: Mathematical models are used to forecast tree and stand growth rates based on current conditions and historical data.
-
Yield Prediction: Analytics predict the amount of timber or biomass that can be harvested in a sustainable manner, allowing forest managers to plan future harvests.
Tools Used: Growth simulators, such as FVS (Forest Vegetation Simulator) or SYMFOR, are used to model forest dynamics under different management scenarios.
2. Sustainability and Carbon Sequestration Analytics
Objective: Monitor forest ecosystems' ability to sequester carbon and their role in mitigating climate change.
-
Carbon Stock Calculations: Analytics calculate how much carbon is stored in a forest (in tree biomass, soil, and dead organic matter).
-
Carbon Sequestration Models: These models estimate how much additional carbon can be sequestered over time as trees grow, based on factors like species, density, and forest age.
-
Sustainability Metrics: Analytical tools assess the impact of forestry practices on long-term ecosystem health, balancing economic output with conservation goals.
Tools Used: Carbon sequestration models like the CBM-CFS3 (Carbon Budget Model) and remote sensing technologies to estimate forest biomass.
3. Biodiversity and Habitat Analytics
Objective: Analyze the impact of forest management practices on biodiversity and wildlife habitats.
-
Species Distribution Modeling: Predicts where certain species are likely to occur based on habitat characteristics and how forest management practices will affect them.
-
Biodiversity Indices: Measures of species richness, evenness, and overall biodiversity are calculated to ensure forest management practices support ecosystem diversity.
-
Habitat Suitability Analysis: Determines how changes in forest structure (e.g., thinning or clear-cutting) affect the quality of habitats for specific wildlife species.
Tools Used: GIS (Geographic Information Systems) for mapping habitat distribution and species data, coupled with biodiversity analytics software like R or EcoSim.
4. Environmental Impact and Risk Analytics
Objective: Assess the environmental impact of forestry activities and manage risks associated with natural disturbances such as fire, pests, and disease.
-
Fire Risk Modeling: Predicts the likelihood of wildfire occurrence based on factors like weather patterns, forest fuel load, and topography.
-
Pest and Disease Analytics: Tracks the spread of forest pests (e.g., bark beetles) or diseases (e.g., fungal infections) and models their impact on tree health and growth.
-
Soil Erosion and Water Quality Analytics: Assesses how forestry practices affect soil stability and water quality in nearby rivers and watersheds.
Tools Used: Tools like FARSITE for fire behavior modeling and LANDIS for simulating ecological processes and disturbance regimes in forests.
5. Remote Sensing and Geospatial Analytics
Objective: Use satellite imagery, aerial surveys, and drones to monitor forest conditions and changes over time.
-
Canopy Cover Analysis: Analyzes satellite or drone imagery to assess forest canopy density and identify gaps due to logging or natural disturbances.
-
Forest Health Monitoring: Remote sensing can detect changes in tree health (e.g., leaf chlorophyll levels, water stress) by analyzing spectral data.
-
Land Use and Land Cover Change (LULC) Analysis: Tracks changes in forest cover over time to assess the impact of urbanization, agriculture, or logging activities.
Tools Used: Remote sensing platforms like Google Earth Engine, Landsat, and drone-based LiDAR (Light Detection and Ranging) technologies to collect and analyze spatial data.
6. Harvest and Financial Analytics
Objective: Optimize the financial performance of timber operations by analyzing harvest schedules, costs, and market conditions.
-
Optimal Harvest Planning: Analyzes the best times to harvest based on timber market prices, growth rates, and forest conditions, ensuring profitability while maintaining forest health.
-
Cost-Benefit Analysis: Assesses the financial returns from different management strategies (e.g., selective cutting vs. clear-cutting) versus the ecological cost.
-
Supply Chain and Market Analytics: Tracks timber supply chain logistics, pricing trends, and demand forecasts to improve the timing of harvests and sales.
Tools Used: Financial modeling tools, ERP (Enterprise Resource Planning) systems for supply chain management, and market forecasting software.
7. Climate and Weather Impact Analytics
Objective: Model the effects of climate change and weather variability on forest growth and resilience.
-
Climate Projections: Analytics combine climate models with forest growth models to forecast how changes in temperature, precipitation, and CO2 levels will impact forest ecosystems.
-
Drought Stress Analysis: Identifies areas of the forest most vulnerable to drought by analyzing soil moisture levels and tree health data.
-
Adaptive Management Scenarios: Models different management approaches (e.g., reforestation, species selection) under varying climate futures to develop resilient forestry strategies.
Tools Used: Climate models like CMIP6 and forest simulation models to project future scenarios.
8. Water Management and Hydrological Analytics
Objective: Assess the effects of forest management on water resources, including watershed health and hydrological cycles.
-
Water Yield Modeling: Estimates the amount of water that flows from forested watersheds into rivers, lakes, or reservoirs, ensuring water supply sustainability for human use and ecosystems.
-
Hydrological Cycle Analysis: Models how forest structure (e.g., tree density, root systems) affects evapotranspiration, infiltration, and groundwater recharge rates.
-
Erosion and Sediment Transport Analysis: Evaluates how logging practices or forest disturbances impact soil erosion and the transport of sediment into water bodies, potentially harming aquatic ecosystems.
Tools Used: Hydrological models like SWAT (Soil and Water Assessment Tool) to simulate the effects of land management on water flow and quality.
9. Socioeconomic Impact Analytics
Objective: Analyze the impact of forestry activities on local communities and the broader economy.
-
Employment and Economic Impact: Tracks job creation in forestry-related activities (e.g., timber harvesting, forest restoration) and estimates the overall economic contribution of forest resources to the local or national economy.
-
Community Benefit Analysis: Assesses how forest management practices impact local communities, including indigenous populations, and their access to forest resources (e.g., fuelwood, non-timber forest products).
-
Recreation and Tourism Analytics: Measures the economic impact of forests on recreational industries (hiking, camping, etc.) and how forest management affects these sectors.
Tools Used: Socioeconomic data analysis software and economic modeling tools to project community-level impacts.
10. Forest Health and Regeneration Analytics
Objective: Assess forest regeneration rates and overall forest health to ensure the long-term sustainability of forest ecosystems.
-
Regeneration Success Metrics: Tracks the success of natural regeneration or reforestation efforts by analyzing tree seedling survival rates, growth patterns, and species diversity.
-
Forest Degradation Monitoring: Analytics detect signs of forest degradation, such as declining tree health, reduced biodiversity, or increased erosion, to intervene before significant damage occurs.
-
Restoration Planning: Combines data on soil health, species composition, and climate conditions to plan effective forest restoration or reforestation projects.
Tools Used: Regeneration models and forest health monitoring systems, often integrated with field data collection tools like mobile apps for foresters.