What are the types of data analysis technologies available?
Below is a continuation of the transcript of the Webinar hosted by InetSoft on the topic of OLAP, the basics of what it is and an explanation of some of the technology terms related to, what OLAP's benefits are, and what the choices in OLAP technologies and OLAP tools are. The speaker is Mark Flaherty, CMO at InetSoft.
Mark Flaherty (MF): When you look at what kinds of data analysis technologies there are in the market, there are two types. They’re based on hardware and software architecture. There is a disk-based and a memory-based solution. And it’s really up to your own requirements for which one might be best suited to you. In some cases there is a hybrid solution combining both of these techniques. And we’ll talk about how InetSoft addresses these techniques.
Essentially the disk-based solution is usually a much more powerful, heavy duty one. It is used with a large data profile, where you are in the terabyte scale of raw data, and you’re looking to do aggregation to take advantage of cubes and summarization. In a lot of cases the disk-based approach requires some data modeling. That can be required in some cases of in-memory solutions, as well. So it’s not necessarily an either-or situation.
#1 Ranking: Read how InetSoft was rated #1 for user adoption in G2's user survey-based index |
|
Read More |
Disk Based Analysis Solution
But in most cases the disk based solution requires some kind of data modeling, so that you can summarize and create aggregations. In a lot of cases disk-based solutions are little bit more inflexible in terms of changing what you’re looking to analyze, what you’re looking to aggregate, et cetera. Most commonly we see a lot of disk based solutions are powered by an OLAP engine. We’ll talk about what OLAP means in the context of data analysis.
In the memory-based environment, data access is much faster, because the information is cached and available through a fast I/O exchange. So you’ve got a very fast, speed-of-thought, kind of interactivity with the data. Whereas in the disk-based model, data access is slower due to the physics of the memory media used.
For in-memory only solutions, usually they are limited to workgroup-sized datasets where workgroups are departments or defined by a dozen or two users. In some cases there might be one terabyte of data used in a workgroup environment or just gigabytes of data. It typically doesn’t get into the multi-terabyte territory which is what we see for a large scale enterprise analytical environment.
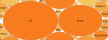 |
Read how InetSoft saves money and resources with deployment flexibility. |
On the Fly Data Modeling
The modeling can be done via a graphical user interface, perhaps with on the fly modeling. Whereas with a disk-based solution, there tends to be more data summarization required to make the environment work properly.
Another feature of in-memory solutions is associative indexing. In some cases, it is defined at the user interface level, meaning I can assign a relationship between tables with a drag and drop tool, or in other cases it is defined automatically through some intelligent techniques built into the query engine. The data source environment can include relational or non-relational data sources, including flat files, spreadsheets, or almost any type of data source. This is another common feature of an in-memory BI solution.
So that is a high-level view of the two types of architectures for analysis, profiles, and products.