Data Science Information Resources
Looking for data science information resources? InetSoft's data science functions are built into its industry pioneering BI platform. Read articles about its capabilities and request a personalized demo.
AI in the Education Field - As the modern-day classroom becomes even more modern with the use of machine learning, AI has spawned a debate on whether this move would benefit everyone or not. First, consider the students: Will they be treated fairly with AI? Or, will AI still be influenced by bias, and be vulnerable to technical flaws. As for teachers, can AI be an effective teaching tool? Or, will AI rob teachers of their ability to teach? With so many questions about AI being used in schools, we would have to consider both sides of the argument: the pros and the cons...
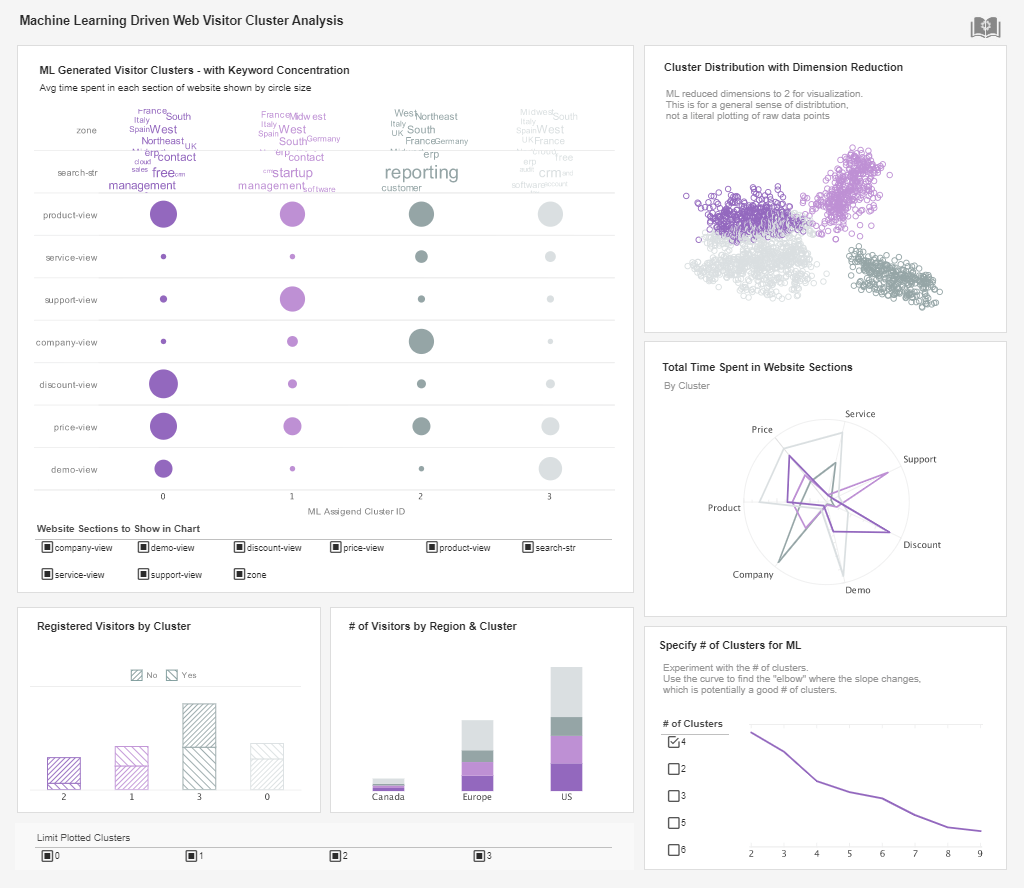 |
Click this screenshot to view a two-minute demo and get an overview of what InetSoft’s BI dashboard reporting software, Style Intelligence, can do and how easy it is to use. |
AI Technologies Can Help the Healthcare Industry - Artificial intelligence (AI) is integral to almost all functions of the healthcare industry covering everything from research and healthcare reporting to predictive analytics for clinical decisions. The role of AI in the healthcare industry is gaining prominence as technology advances and its effect on quality and service delivery is immense. Machines in hospitals, laboratories, and pharmaceutical factories can perform tasks previously done by human labor faster, with precision, and at a fraction of the cost. Here is a brief look at some of the areas AI technologies will improve in healthcare...
Artificial Intelligence Powering Industry Applications - A lot of entrepreneurs still don't realize it, but Artificial Intelligence (AI) is already alive and powering the 21st century businesses. Smart systems make a substantial impact on business operations globally, which turns AI into the biggest driver of lead, sales, and revenue generation. A report reveals that 80% of emerging technologies will have AI foundations by 2021. In such circumstances, it's not surprising to see that the AI industry will be earning $118 billion a year by 2025. When business professionals see stats like the ones we mentioned above, they usually ask a couple of questions: What makes AI so powerful, and does it have any real-life applications already? If you want to see the answers, keep reading this article as we are about to show you practical examples of AI projects in use today...
Content Marketers Use AI and Machine Learning - Content creation in marketing has traditionally been perceived as a creative channel that has almost nothing to do with technology, but the truth is that AI and machine learning are making a profound impact on modern content strategies. Today, it's hard to imagine a productive team of content creators without a whole set of advanced tools and platforms. A recent report suggests that 63% of companies are outperforming competitors with help from marketing automation. But how AI and machine learning are changing the content industry? They do it in many different ways thanks to the sheer versatility of the two technologies. In this article, you will learn the following: The basics of AI and machine learning 9 ways they amplify content marketing strategies...
Data Science Analytic Software Provider - Looking for good data science analytic software providers? InetSoft is a pioneer in self-service analytics whose platform also has built-in machine learning functions. View a demo and try interactive examples...
Data Science Analytics Company - As a data science analytics company, we tend to focus on automation, time savings, and something that we are going to talk about in more detail later, graphical interfaces that can bring people who know more about the business closer to the data. Proprietary solutions also tend to focus on the deployment of models. We were talking about one sort of workflow which when the data scientist develops the model and then hands it off to a programmer to deploy the model. Well, we see InetSoft and other proprietary solutions working towards a one click sort of deploy button, and what a time savings that can be for an organization. What we want to look for and things that we've done is how do we feed the creativity of data scientists, and we think that allowing for bi-directional integration with open source products is one way. I can be in InetSoft, and make calls out to Open Source. Then we're talking about things like using the open standard of a PMML. I think it's really silly, and I do see less and less of this, thank God, people debating is R better than Python better than InetSoft? These are not productive discussions, I don't think. I mean I think it's good to know which tool to use for what. I certainly think that's good. I teach a data mining class and where I expose this to my students. Know which tool saves you the most time at what point of the process. I think that's more important...
Data Science and Data Scientists - I have heard a lot lately about data scientists. It seems like these guys are almost as big as Justin Bieber right now. I even saw the Harvard Business Review recently called data scientist the sexiest job of the 21st century. Is that cool or what? When I saw that article, and I had to tweet "that true, if they disqualified actors, singers, dancers and beauty queens from the contest, then yes it's probably on the top 10 sexiest jobs". But you know my criterion for sexiest job is that the people have got to look good naked. I doubt that data scientists fill that bill, but you never know. But no we don't want them tweeting those pictures to us right now. But let's get serious for a second. What is a data scientist? I keep getting asked that question for the good reason that I keep a blogging on the topic and talking about it constantly. The whole notion of a data scientist is that it is somebody who is an analytics professional, whose core job it is to build statistical models of complex data sets, large complex data sets, in order to be able to find statistical patterns within that data that are not apparent to the naked eye or may not be apparent to structured reports that let's say you might pull up in your business intelligence application. So we can say statistical modeling, fundamentally, it's what a data scientist does. And they build statistical models for a number of purposes. Applications and businesses have been using them for a long time...
Data Science Dashboard Tool - Are you looking for a good dashboard tool to display your data science model results? InetSoft's pioneering dashboard application makes producing great-looking web-based dashboards easy with a drag-and-drop designer and the ability to connect to all types of big data sources. You can also connect it to R ML models and visualize the results of changing feature inputs dynamically. View a demo and try interactive examples...
Data Science and Healthcare Data Analysis - So what's been the common thread from cyber security to healthcare in terms of analytics and data science? What are the technical and other hurdles that are common between them that allowed you to make that leap? So the ability to detect various kinds of events in your data streams, and I'm going to use that term somewhat loosely, detecting events and data streams and then combining them together into patterns is something that happens in cyber security as well as fraud, waste and abuse. So whether you do that by looking at a series of bad claims or a series of services that shouldn't have occurred in sequence is a simple idea. We can't pull a tooth from a baby. That's a great example of a very simple idea, that sequence of events shouldn't be happening in healthcare, and you can see in a series of network activity the same kinds of events in principle would occur as a series of patterns and as you get good at detecting those kinds of patterns you can apply the same skill and learning about how to deal with the 3Vs of big data, the variety and the velocity and whatnot to really harness this in very high speed fashion. Right, now not that the cyber security issues aren't important, but are the stakes higher for healthcare, or is the market larger? What's at stake when you point your technology and expertise at the healthcare sector...
#1 Ranking: Read how InetSoft was rated #1 for user adoption in G2's user survey-based index |
|
Read More |
Data Science-Intensive Work - You need a data scientist to help your company make sense of big data. While a data scientist may have been involved in the selection of statistical methods and algorithms that power your data sources, data scientists are not necessarily needed to make sense of big data. Once a business intelligence solution has been designed, tested, and implemented, it should be readily accessible to most information workers. Much of the data science-intensive work has already been done. Leave the computer and data science to the business intelligence and big data solution developers and invest in a system that's ready to run right out of the box. Modules, mashups, dashboards, and other built-in tools definitely required a data scientist to help develop them, but, if they're designed properly, they will not require a data scientist to hold your hand while you use them. If they do, it's time to look for new big data and business intelligence solutions...
Data Science Posts - What data is needed for machine learning tools to detect and predict churn? This post is the second in a series discussing a machine learning use case for a mobile app provider. Why Data Science is Important When opening a business magazine or reading a news article about business trends, one is becoming more and more...
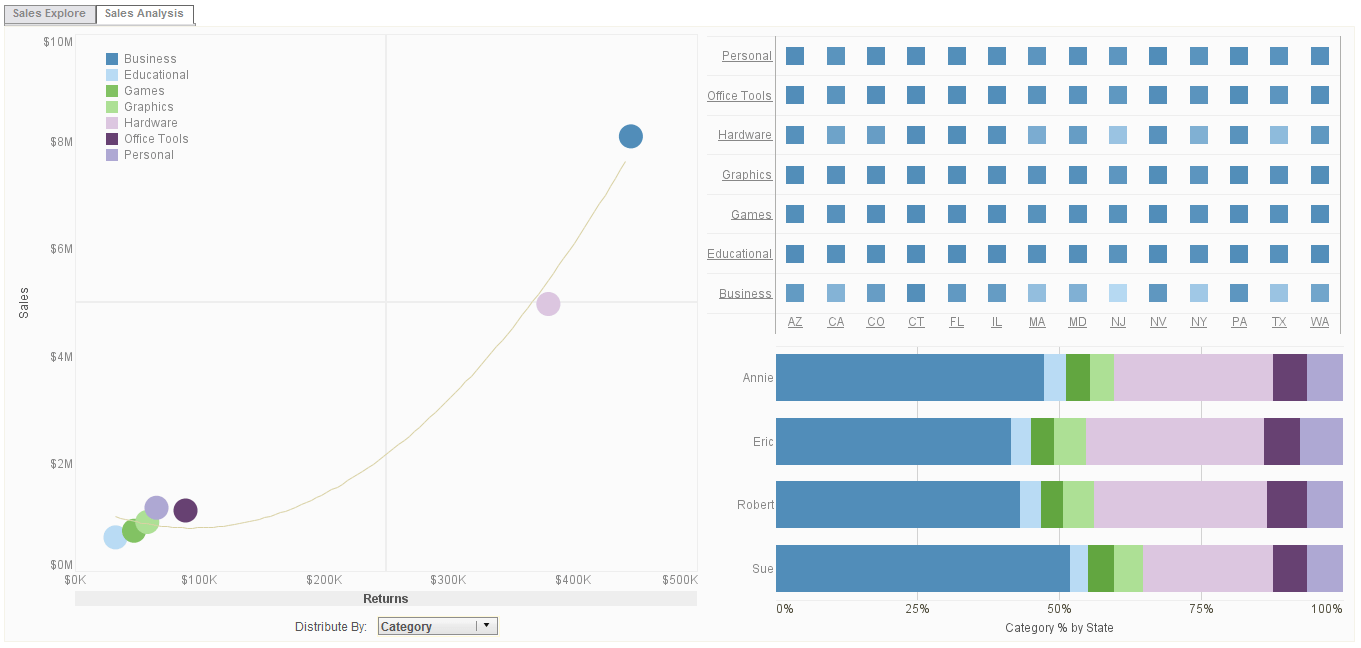 |
Click this screenshot to view a two-minute demo and get an overview of what InetSoft’s BI dashboard reporting software, Style Intelligence, can do and how easy it is to use. |
Data Science Reporting Visualization Tools - Looking for a good reporting visualization tools for data science? InetSoft is pioneering BI platform offers analytics for ML models built in Spark. View a demo and try interactive examples...
Data Scientists Create Algorithms - Applications of artificial intelligence range widely from the banking and finance sector to medical care, information technology, agriculture, and even human resource management. Data scientists create algorithms and predictive analysis of the behavior of machines. They install a set of instructions to perform specific tasks. As the machine is programmed and strictly goes by the logic of algorithms, there is little to no chance of any mistakes. That's why AI is used for the safety and security facilities for facial recognition. The ultimate goal of scientists is to provide AI systems with problem-solving and decision making capability. Although the dream is far from reality, people are still afraid that AI robots might take over the world or individuals will be unemployed in the future because a robot replaced them...
Data Scientists and Domain Experts - When we talk about the current machine learning adoption trends, are we expecting data scientists to be domain experts, to be business domain experts? Is that a realistic expectation? I think that there's this myth of the 23-year old data scientist who knows about math, knows about computers and knows about business. I think there's plenty of really smart, super sharp people out there, young people out there who may really know math and statistics and really may know their computer hacking skills extremely well, but that substantive expertise can take years to develop. I think there's a little - if we are lumping business expertise into the data scientist definition, then I think that takes some time to cultivate for sure. Some problems that we see with data scientists and managing data scientists is sometimes if this is a new function in an organization, management might not really understand how they need to work. One classic pitfall is getting bogged down with algorithms. Spending all your time picking the best algorithm or trying to tune algorithms instead of just focusing on solving the business problem, instead of focusing on the fastest way to solve the business problem. There can be all kinds of issues between data science and IT, who owns the data, who owns the tools, who owns the hardware that can cause conflict. Let's get onto automation because it's a topic that I like to speak very passionately about to data scientists but also to organizations because I think it's really important. There are a lot of people on this WebEx today. There's a lot of interest out there for what data science, and that is very encouraging. Still, within any individual organization there's always more demand than the actual data scientist can fill...
Definition of Decision AI - It's not just about personal assistants or autonomous vehicles; artificial intelligence has fundamentally changed how we live and work. Nowadays, businesses and organizations from a variety of industries use AI in their decision-making procedures. Artificial intelligence's subfield known as Decision AI, also known as Decision-making AI, aids in automating decision-making procedures. We'll delve deeper into what Decision AI is and how it functions in this article. The Definition of Decision AI Decision AI is the application of AI algorithms to assist humans in making decisions or to make decisions on their behalf. Numerous industries, including healthcare, finance, logistics, and others, can use this technology. Machine learning, data analytics, and natural language processing are all combined in Decision AI. It gathers information from numerous sources, analyzes it, and then provides insights and suggestions for action to decision-makers...
Defining Data Science Relative To Analytics - Thank you all for joining us today. I'm Abhishek Gupta, I am the Chief Data Scientist at InetSoft. We have four major points that we want to discuss today. The first one being the importance of data science and data scientists and bringing machine learning into organizations. The second one being we've all heard of the V's of big data, and we know that one is velocity, and we know that there's a lot of streaming data out there now. I feel like that's going to be a big part of organizational strategies moving forward. Point three, how an organization can keep creativity with machine learning. We have all of these different tools to choose from today, all of this different data, but we deal with regulation. We deal with documentation. We deal with productionizaling machine learning code. How do we keep infusing creativity into the machine learning workflow within an organization. Then, we've also heard a lot about the citizen data scientist recently, and just in general more and more people in organizations wanting to get involve with analytics and machine learning. So that's point four. Okay, so we're going to start our discussion here, is any of this really new? Is machine learning new? Is data science new? To me this is resounding no. In fact, machine learning has been studied at least since the 1950s, maybe before. Data science you could say goes back to John Tukey's 1962 Future of Data Analysis Paper. There's a great recent paper by Donoho out of Stanford that talks about 50 years of history of data science, and I urge you to read that...
Deploying a Data Science Solution - Sure, and as we're looking to the future, not only on the payoffs and the implications of where this is relevant, there's also some architectural changes with the way people are using a hybrid cloud technologies sourcing their data centers in different ways. Tell us a little bit about how you're deploying your data science solution. Are you using this all on premise? Do your security requirements make that necessary now? Are you into a hybrid mode, or how will that pan out in the future, and how might that impact how you can get more data into a compute mode that you can analyze? Jim: So, over time there's no doubt that the hybrid or deployment models were some of it will be on premise, and some of it will be in the cloud. This is going to happen in healthcare and healthcare claims, but still as people are seeing in the news, there is still a lot of apprehension around doing healthcare in the cloud, and so a lot of our requirements require us to keep data under lock and key. Over time as things get more secure, there's no doubt that this hybrid cloud and on premise deployment is going to happen and building an architecture that allows you to segment your data where you can discriminate the things that go out into the cloud versus things that you have to keep private is something that should be top of mind in any effort of this size or scale...
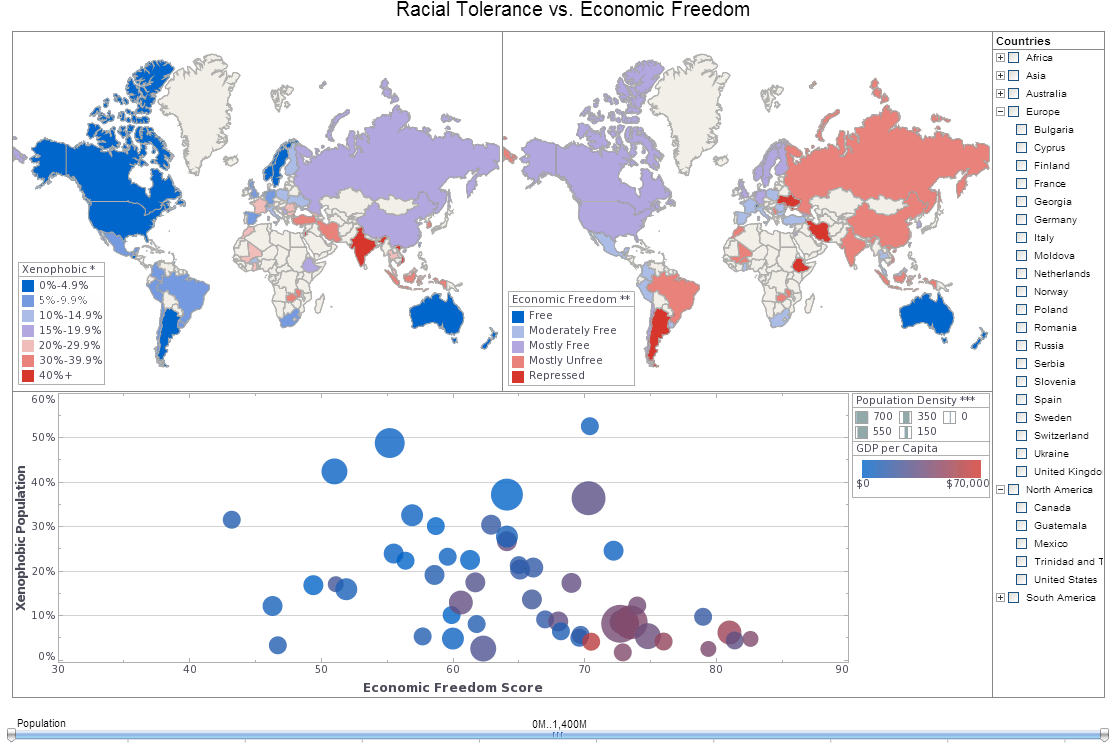 |
Click this screenshot to view a two-minute demo and get an overview of what InetSoft’s BI dashboard reporting software, Style Intelligence, can do and how easy it is to use. |
Different Types of Data Scientists - All right, so let's focus on this data scientist persona and how that is affecting machine learning in organizations. Well, we definitely believe that machine learning is being taken more seriously as data science and data scientists are taking more seriously. There's a lot to unpack here, but we've seen over the past few years sort of a segmentation of data scientist where there's different types of data scientists, different levels of data scientists. This is important as you develop your machine learning strategy in your organization to realize that there are some data scientists who truly need to be given a lot of freedom, access to a lot of data, access to a lot of different tools, freedom within the organization, whereas other data scientists, more junior data scientists need more supervision and need more guidance and possibly need tools that can help them out more...
Different Types of Data Scientists - Another thing that we hear about a lot is the unicorn, and I think these unicorns do exist. They're hard to find. They're hard to keep, but if you can get one, good for you. If not, a lot of people are looking to build teams from preexisting resources and talent in their organization. I think that's a great thing to do. We're also looking at a Data Science Venn Diagram, and I think Another thing that we hear about a lot is the unicorn, and I think these unicorns do exist. They're hard to find. They're hard to keep, but if you can get one, good for you. If not, a lot of people are looking to build teams from preexisting resources and talent in their organization. I think that's a great thing to do. We're also looking at a Data Science Venn Diagram, and I think most organizations could dig up some programmers and statisticians and some business people and put them on a team together and that kind of equals a data scientist...
Do Auditors Need to Be Data Scientists? - While auditors don't necessarily need to be data scientists, they do need to have a strong understanding of data analysis and be able to use data analytics tools effectively. In today's business environment, auditors are faced with increasingly complex data sets that require advanced analytical skills to properly assess. To effectively audit these data sets, auditors need to be able to identify relevant data points, analyze data, and draw meaningful insights from it. While some auditors may have the requisite skills to do this themselves, others may rely on specialists or technology tools to help them perform these tasks. Data science is a specialized field that requires advanced skills in statistics, programming, and machine learning, among other areas. While some auditors may have these skills, it's not typically a requirement for the profession. However, as data analysis becomes an increasingly important aspect of auditing, auditors who are proficient in data science may have a competitive advantage over those who aren't...
Do I Need to Hire a Dozen Data Scientists - A couple weeks ago in "Justifying a BI Purchase", we made the case of how the right BI tool is a worthwhile purchase even for organization's that are struggling to get into the black, ones who don't think they have the budget for BI. Last week, DATAVERSITY addressed another budgetary concern related to implementing BI, "Can My Business Achieve Optimal Analytics Without Hiring Dozens of Data Scientists?". In addition to addressing a concern put forth by many organizations who are just starting to get their feet wet with data science and analytics, the article underscores the importance of bringing self service BI to nontechnical business users, a philosophy that we at InetSoft have been promoting for the better part of two decades. "In this rapidly changing, increasingly competitive business landscape, the wise enterprise will focus, not on adding dozens of data scientists, but on capitalizing on the time and skills of those data scientists and analysts by giving business users the tools they need to make the day-to-day decisions and produce data-driven analytics that are accurate and timely without the assistance of a data scientist…
Example of AI Being Used in Proactive Intervention - One compelling example of AI being used in proactive intervention is in healthcare, specifically in the field of predictive analytics for patient care. Hospitals and healthcare systems are increasingly leveraging AI algorithms to analyze vast amounts of patient data, including electronic health records (EHRs), medical imaging, lab results, and vital signs, to identify patterns and trends that could signal potential health risks or adverse outcomes. By applying machine learning and predictive modeling techniques to this data, healthcare providers can proactively intervene to prevent or mitigate health issues before they escalate. For instance, AI algorithms can analyze historical patient data to predict the likelihood of readmission within a certain timeframe for specific patient populations, such as those with chronic diseases or undergoing certain medical procedures. By identifying patients at high risk of readmission, healthcare providers can implement targeted interventions, such as personalized care plans, medication adjustments, or follow-up appointments, to prevent readmissions and improve patient outcomes. These proactive interventions not only enhance patient care but also reduce healthcare costs associated with avoidable hospitalizations and complications...
Example of Data Science Applied to Healthcare - How about an example of data science applied to healthcare? Do we have an opportunity to look to beyond the technology into an actual use case? You probably can't name them, but where and how is this being used, and what are the paybacks? So, we work in large, whether that'd be private or public, and we will deal with something in the size of a Medicaid program for a state. So when you imagine the population of a state and the number of people involved in a healthcare program of that size, we have to analyze the entire program and all the claims and recipients and providers in that healthcare program. So that data is really large. Abhishek: So provide an example perhaps as to what was the case before and then after applying your data science solution to the problem. Jim: So the biggest challenge that most of them face is which I alluded to earlier is that they don't need to go out and figure out in this big data set of claims what do I have to do today to actually help solve the problem of doing recruitments or teaching people how to bill more properly, or getting rid of waste or abuse, or asking for money back, or changing policy so that people stop paying for things that shouldn't be paid for...