Machine Intelligence Solution Resources
Find machine intelligence solution resources from InetSoft, an industry pioneering providing easier, more cost-effective business software solutions. Request a personalized demo.
Five Amazing Machine Learning Examples You Should Know About - The current state of machine learning is that adoption by businesses has dramatically increased in 2018 compared to 2017. This increased adoption is in line with Deloitte Global's 2018 machine learning report, which predicted that machine learning implementations would double in 2018 from the previous year. The same report projects implementations to double again by 2020. However, there is a shortfall in terms of the number of machine learning implementations at individual organizations as CEOs and business executives try to define and understand the particular business benefits that these algorithms drive. Advancements such as automated data exploration and the use of "synthetic" algorithmically generated data are driving surges in machine learning adoption as it becomes less difficult, less costly, and less time-consuming to run machine learning projects. The point on synthetic data is particularly important considering how training a machine learning model typically requires millions of labeled data elements, which is costly and takes a significant amount of time. The synthetic data mimics the characteristics of real-world training data without the same difficulty in acquiring the data. Another technique with excellent potential to decrease the number of examples you need to feed into a machine learning model is the idea of transfer learning. The basic premise here is that you pre-train a model to perform one task and re-use the same model as the basis for perform a second, related task in a similar domain. For example, a model that can recognize cats might be re-used to recognize tigers...
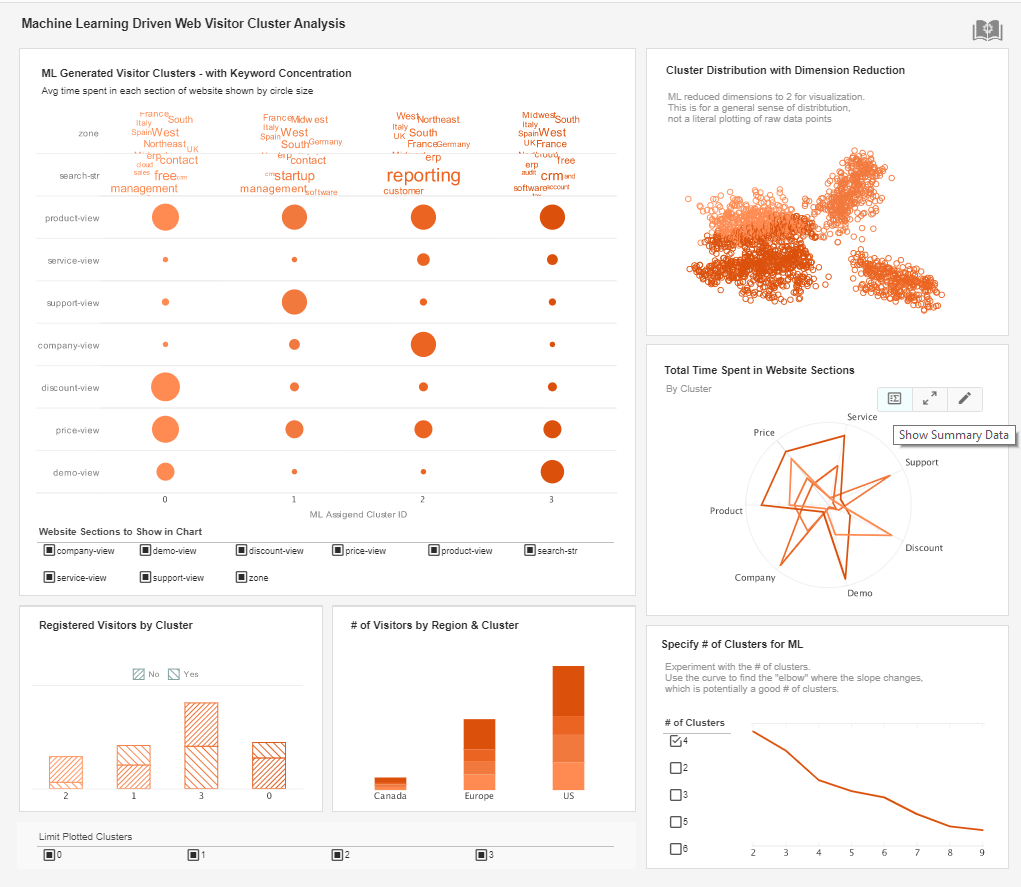 |
Click this screenshot to view a two-minute demo and get an overview of what InetSoft’s BI dashboard reporting software, Style Intelligence, can do and how easy it is to use. |
Future of Machine Learning Applications in Education - When most students hear about machine learning, they believe that it means that there is no teacher or some alien technology involved. In truth, things are more prosaic as the education system has only started implementing innovative machine solutions in practice. There are online video lessons and automatic guides that help students proceed through the course, yet machine learning goes further than that. It also stands for mobile learning applications, video surveillance systems, and artificial intelligence, which are used on a daily basis. The future of such an approach is most likely to develop in terms of learning assistance and providing help akin to what a physical teacher offers. It will always evolve according to human needs, yet it should not be seen as a substitute for existing practices or an actual psycho-social interaction between educators and students. The key is to implement machine learning wisely and study the benefits...
Hospital Use Case of Machine Learning - As covered by tech news site Futurism (click here for the article) the Children's Hospital of Philadelphia has successfully trained machine learning algorithms to identify cases of sepsis in newborns significantly faster, greatly increasing the infants chance of survival. "Using electronic health record data, such as vital signs like blood pressure and temperature, from 618 infants in the CHOP neonatal intensive care unit from 2014 to 2017, the team trained eight machine-learning models to compare vital signs to 36 potential indicators of infant sepsis. Because the data was retroactive, the research team was able to compare the machine-learning models' accuracy to clinical findings. Of the eight models, six were able to accurately identify cases of sepsis up to four hours earlier than clinicians had." Weighing 36 potential indicators is just what machine learning was created for: tasks that require the type of pattern recognition that traditional computers lack, recognizing patterns at a level of complexity that human brains are incapable of processing. Having a machine learning model that you can repeatedly train to make better predictions is the equivalent of having a data scientist with a genius level IQ who is constantly experimenting and writing better predictive models for your organization. Curious as to how these ML models can be integrated with interactive visualizations and automated reporting? Click on the image below to open an InetSoft Customer Churn dashboard which includes a trainable predictive model of customer churn factors...
How Does a Legacy System Affect the Process of Setting Up Machine Learning Solution - One last question which is probably on the minds of many of our listeners is how does a legacy system affect the process of implementing machine learning solutions? That's a question that I get a lot from our installed base. InetSoft's has chosen the a route of making all machine learning solutions technically available as add-on services running either on premise or in the cloud. With our API and multiple integration points, we're able to enable a rigorous integration of machine learning capabilities into existing architectures without the need to be on the fully latest version number of every single product. I think this is something that all customers look for and appreciate because it helps them drive business value today while also embarking on longer term harmonization and then making an upgrade journey in parallel. So now I'd like to just reiterate a few of the main points as we bring this webinar to a conclusion. Artificial intelligence is enabling us to develop intelligent machines that are helping to enhance our human capabilities and further enabling enterprises to succeed. What we've seen in all the examples today is how machine learning has allowed businesses to reimagine their business processes and to reimagine all the applications of ML...
#1 Ranking: Read how InetSoft was rated #1 for user adoption in G2's user survey-based index |
|
Read More |
How We Can Get Started Implementing A Machine Learning Solution - This brings us to the next point about how we can get started implementing machine learning solutions. First I think we've seen increasing machine learning processing power that's really required, and it enables you to increase your computing power. Additionally enterprises can implement machine learning when they're dealing with very specific scenarios and very specific conditions. We were discussing already a ton of examples for this. Machine learning is able to make predictions on new data and automate repetitive tasks like the support ticket classification I mentioned earlier, which is a good way to start. We see also enterprises need to properly prepare the data by minimizing their information silos and developing a real time modern data analytics infrastructure. Now we see many organizations are organized by department and have data silos. They need to integrate the data from all the different sources, such as customers and their supplier sources because otherwise you cannot fully use the algorithms if you don't have the relevant data and if you don't have the right quality of data. I think what we see as well is a movement towards the cloud for data storage. To have your data all in the cloud in order to process these high volumes of data that are integrated from all various sources. Moreover, enterprises need to align, and this I think is very important because it's not only technology. They need to align their people, the processes and the technology to create a different and a better organizational foundation that supports this digital core and data driven thinking...
How Machine Learning Is Changing Business Analytics - Thank you all for joining us today for a discussion about how machine learning is changing business analytics. We have four major points that we want to discuss today. The first one being the importance of data science and data scientist and bringing machine learning into organizations. The second one being we've all heard of the V's of big data. and we know that one is velocity, and we know that there's a lot of streaming data out there now. This is going to be a big part of organizational strategies moving forward. Point three, how an organization can keep creativity with machine learning. We have all of these different tools to choose from today, all of this different data, but we deal with regulation, we deal with documentation, we deal with productionizing code. How do we keep infusing creativity into the machine learning workflow within an organization? Then, we've also heard a lot about the citizen data scientist recently and just in general more and more people in organizations wanting to get involved with analytics and machine learning, so that's point four. Okay, so we're going to start our discussion here. Is any of this really new? Is machine learning new? Is data science new? To me this is resounding no. In fact, machine learning has been studied at least since the 1950s, maybe before. Data science you could say goes back to John Tukey's 1962 Future of Data Analysis Paper. There's a great recent paper by David Donoho out of Stanford that talks about 50 years of history of data science, and I urge you to read that. We have a link to that at the end. We're seeing machine learning in organizations now. This isn't coming out of the blue. This has a long history, and so we wanted to spend a little bit of time here. One good thing to do at first is of course to define machine learning, and that's really tricky. I think for better or for worse, in a certain sense machine learning has taken on sort of a pop culture, meaning it's just the rebranding of analytics or data mining...
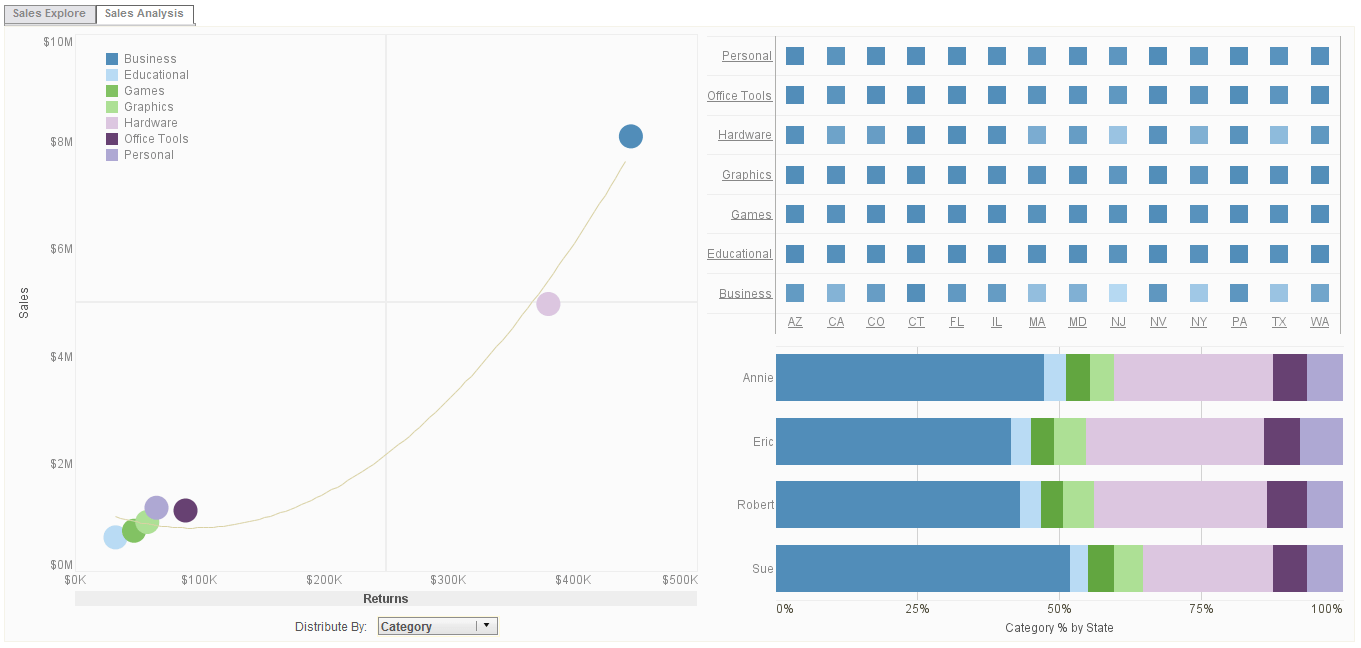 |
Click this screenshot to view a two-minute demo and get an overview of what InetSoft’s BI dashboard reporting software, Style Intelligence, can do and how easy it is to use. |
How Machine Learning is Helping Personalize Travel Insurance Offers - In yet another example of how machine learning is being used to help humans make better decisions, Allianz Travel Insurance has upgraded its online platform with added personalization based on machine-learning and artificial intelligence technology to deliver better travel insurance policy recommendations, fighting the perception that a travel insurance partner is a poor place to buy an insurance policy. This new platform selects from 100 different offerings in a matter of seconds, and gets more effective with customer use. According to a recent article in Forbes: "The technology behind this customization is impressive. Allianz collects anonymized information to power a personalization engine from a partner like Priceline to help it find the right product for the customer. That reduces the time spent researching companies and products and virtually eliminates the chance that customers will pay for coverage they don't need… The engine itself takes multiple trip and traveler attributes into consideration, selecting from about 100 different products. What's more, the personalization engine gets smarter every day." The utility demonstrated here underscores how machine learning can make decisions based on far more factors than a human mind can process at once. Just a few of the factors that the system takes into account are: customer service hours self-managed vs outsourced travel assistance services in house medical team vs outsourced trip length connecting flights trip cost group size age of travelers reasons for travels connecting flights timing of trip This type of complex decision making enabled by machine learning algorithms is now available to InetSoft customers, incorporated alongside interactive visualizations, striking a balance between human and machine generated decisions. InetSoft's flexible data layer also makes incorporating even off premise data (such as the anonymized customer data from a third party provider in this example) easy and intuitive. For example, the InetSoft sample dashboard pictured below displays flight delays across the United States in real-time, by continuously pulling the data from the FAA website. Click on the screenshot below to open up the live dashboard. The data is accurate and up to date, so you can even use this dashboard to check and see if your flight is ontime...
How to Use AI Machine Learning Software in Your Business - To many people, AI and Machine Learning are only concepts, things of the distant future, something that we have to wait years for. However, the truth is that we're already on the verge of AI revolution, and the increase in the ease of use of machine learning software has been rapidly increasing its impact in a number of industries. From simple AI-powered chatbots using natural language processing technology to complex autonomous driving systems and warehouse management systems able to deliver a huge increase in efficiency and effectiveness, the world has been using AI-powered tools for quite a while now. For businesses, AI and machine learning software represents a great opportunity to gain competitive advantage, increase sales, and remain relevant for years to come. Many well-known companies are already using machine learning to achieve their goals; for example, DHL deploys it to increase effectiveness and efficiency of their logistics chains and warehouse management, UPS uses machine learning to determine the fastest routes for drivers, and Tesla has AI-powered platforms for self-driving cars. In other words, the future is much closer than you think. "Adopting AI to improve operations is something that you have to do as soon as possible to become an early adopter and outperform your competition," recommends Allison Borders, a digital business advisor at Collegepaper. Here are some potential uses of machine learning software to advance your business and meet the challenges of the future...
Is a machine learning platform useful for a small dataset - This post is the third in a series discussing a machine learning use case for a mobile app provider. The link to the full case study can be found at the end of the post. The first post can be found at https://www.inetsoft.com/blog/machine-learning-concepts-defining-churn-predictive-metrics/ Is machine learning useful for a small dataset? Machine learning is not associated with data volume. The most widely known dataset for machine learning is call "iris". This is a dataset for three species of iris flowers. It only contains 150 rows (observations) of data with 5 columns (features). This dataset has been used to test and validate many machine learning algorithms and models. In the business world, it is very common to see a project start with a spreadsheet of a few thousands row of data. Data in such a spreadsheet is normally already aggregated and transformed like in the use case we have been discussing. Sometimes in business applications, the challenge is about the number of features (columns). Too many features will require a lot more computational power and make the process slow. It can also make the results harder to understand. That's where business expertise is needed to choose the right set of features, at least initially...
Inspiration for Artificial Neural Networks - In this article, continuing our introduction to machine learning, I am going to write a little bit about real neurons and the real brain which provide the inspiration for the artificial neural networks that we are striving to learn about in this series of articles. In most of the discussion, we won't talk much about real neurons, but I wanted to give you a quick overview at the beginning. There are several different reasons to study how networks of neurons can compute things. The first is to understand how the brain actually works. You might think we could do just by experiments on the brain, but it's very big and complicated, and it dies when you poke around it too much. And so we need to use computer simulations to help us understand what we are discovering in empirical studies. The second reason is to understand a style of parallel computation that's inspired by the fact that the brain can compute with a big parallel network available from real neurons. If we can understand that style of parallel computation we might be able to make better parallel computers...
Inspiring machine learning applications & examples - It's clear that machine learning technology is an invaluable data-driven tool that expands business intelligence across the entire organization. To put this notion into perspective, here are two inspiring examples of machine learning in action. As one of the world's most pioneering machine learning innovations, IBM Watson is a business intelligence system responsible for helping a number of leading brands exceed their commercial potential in recent years. A supercomputer that merges ML with cutting-edge analytical software, IBM Watson is essentially a smart question-answering application that processes information at a rate of 80 teraflops (a trillion floating-point operations per second)...
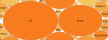 |
Read how InetSoft saves money and resources with deployment flexibility. |
Intro to Data Visualization and Machine Learning - In this digital era where troves of data are being collected every second, it is important to utilize tools and techniques that help understand this data if it is to inform better business decisions. Machine learning is a branch of artificial intelligence that is being widely used in analyzing data using algorithms to learn from the data. It then makes intelligent suggestions and predictions based on the data collected. Integrating data visualization with this data analysis technique brings in a suite of tools that help in finding a qualitative understanding of these massive amounts of data. Read on to understand how tapping into the possibilities of machine learning data visualization is important in improving data analytics...
Intro to Machine Learning from Our CTO - Our CTO recently wrote up an introduction to analytics and machine learning to help people who might be knowledgeable about traditional dashboarding reporting software and analytics but have only heard of the term "machine learning." The not-too-long article helps give examples of how it can be applied in various business settings, and of course, if it inspires you to start mining your own data to develop automated decisioning to make your organization higher-performing or more efficient, we can help...
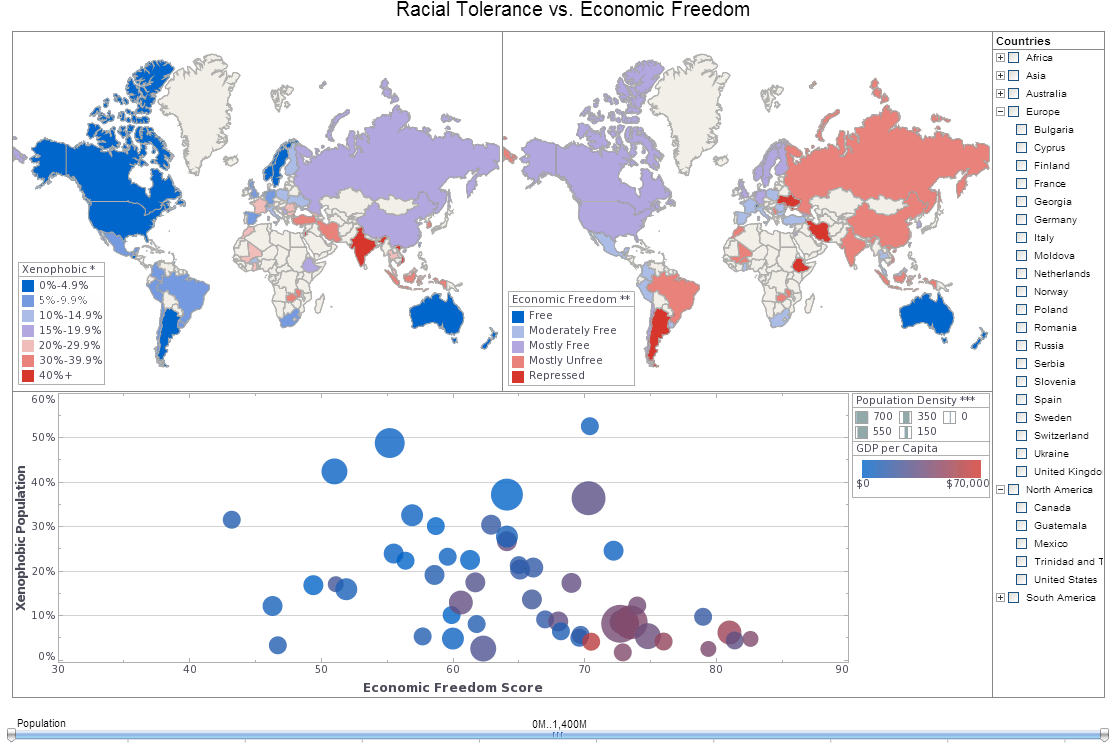 |
Click this screenshot to view a two-minute demo and get an overview of what InetSoft’s BI dashboard reporting software, Style Intelligence, can do and how easy it is to use. |
Introduction to Machine Learning and Some Examples - Today I wanted to write an article to talk about a topic of growing popularity in the business intelligence market: machine learning. Why do we need machine learning? What are the kinds of things we use it for? What are some examples of what machine learning can do? The reason we need machine learning is that there are some problems where it's very hard to write software program to solve. Take recognizing a three-dimensional object, for example. When it's from a novel viewpoint in new lighting conditions in a cluttered scene, it is very hard to do for a non-human system. We didn't know what program to write because we didn't know how it's done in our brain. And even if we did know what program to write it might be that it was a horrendously complicated program. Another example is detecting a fraudulent credit card transaction where there may not be any nice simple rules that will tell you it's fraudulent. You would need to combine a very large number of not very reliable rules, and also those rules change over time because people change the tricks they use for fraud. So we need a complicated program that combines unreliable rules, and those rules need to be changed easily...
Machine Learning Algorithm Primer for Non-Techies: Number 1 – Learning by Example - This post is the sixth in a series discussing machine learning examples for a mobile app provider. The link to the full case study can be found at the end of the post. The first post can be found at https://www.inetsoft.com/blog/machine-learning-concepts-defining-churn-predictive-metrics/ Machine Learning Algorithm Primer for Non-Techies: Number 1 – Learning by Example Learning by example is also often called supervised learning. Supervised means the machine learning algorithm learns from examples of correct predictions given to it by a human. Once learned, the model can take an input (observation), examine its attributes (features), and output a new attribute for the observation. There are two types of supervised learning: classification and regression. Classification is the type illustrated in the churn prediction use case that we have been discussing. In the classification model, a user is , assigned a predefined label (churn or no-churn). There many different classification algorithms. Some are very simple, like naïve Bayes. A simple algorithm runs fast and surprisingly outperforms complex ones in many real world cases. Here is a list of common classification algorithms: linear SVMs (support vector machines) logistic regression decision trees random forests gradient-boosted trees naive Bayes Regression is the second type of supervised learning. Unlike classification, the output for a regression algorithm is a numeric value. For example, given a person's height and other features predict the person's weight. Common regression algorithms include..
Machine Learning Algorithm Primer for Non-Techies, Number 2: Machine Discoveries - This post is the seventh in a series discussing a machine learning use case for a mobile app provider. The link to the full case study can be found at the end of the post. The first post can be found at https://www.inetsoft.com/blog/machine-learning-concepts-defining-churn-predictive-metrics/ Unsupervised learning, unlike supervised learning, asks machine learning to find patterns on its own. In this method, no human provides examples to be used as a base to learn from. For business applications, two type of unsupervised learning are often seen: clustering and association. Clustering classifies observations into categories called clusters. But unlike classification, there are no predefined categories. Machine learning concepts try to put similar observations into a cluster. For example, you might want to see how many clusters your customers belong to. The hope is that there will be things that are in common with people in a cluster so you can market to them with a message that is specific and meaningful in one cluster differently from how you do so for another cluster. You can experiment to see how many clusters make most sense for the data. Judgment is necessary to determine whether the clusters do define a group of people with meaningful differences from other clusters.. Common clustering algorithms include: K-means Gaussian mixture Power iteration clustering (PIC) Latent Dirichlet allocation (LDA) Bisecting k-means Streaming k-means Association finds things that are related. You may have heard of Frequent Pattern Mining, Market Basket Analysis and so on. These are all refer to association type of algorithms. In machine learning jargon, this is most often called Collaborative Filtering. This is because a user's potential interest can be found by examining other users' interests who already share some common interest with the target user. An example is product recommendation where products that are frequently bought together are associated. Note that association learns from past sales data to find the relationship. But this past data set is simply raw collected sales data, not something that a human has scored or assigned. Algorithms include: Memory based collaborative filtering Model based collaborative filtering Association Rules...
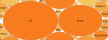 |
Read how InetSoft saves money and resources with deployment flexibility. |
Machine Learning Analytic Software Provider - Looking for machine learning analytic software providers? InetSoft is a pioneer in self-service analytics, and our solution lets data scientists and business users visulize model results to see which features are driving predictions. View a demo and try interactive examples...
Machine Learning Analytics Alternative forDatabricks - Are you looking for a good alternative to Databricks dashboard software? InetSoft's pioneering dashboard reporting application produces great-looking web-based dashboards with an easy-to-use drag-and-drop designer. Mashup your databricks data with any other in your organization. Get cloud-flexibility for your deployment. Minimize costs with a small-footprint solution. Maximize self-service for all types of users. No dedicated BI developer required. View a demo and try interactive examples...
Machine Learning Analytics Company - As a machine learning analytics company, we tend to focus on automation, time savings, and something that we are going to talk about in more detail later, graphical interfaces that can bring people who know more about the business closer to the data. Proprietary solutions also tend to focus on the deployment of models. We were talking about one sort of workflow which when the data scientist develops the model and then hands it off to a programmer to deploy the model. Well, we see InetSoft and other proprietary solutions working towards a one click sort of deploy button, and what a time savings that can be for an organization. What we want to look for and things that we've done is how do we feed the creativity of data scientists, and we think that allowing for bi-directional integration with open source products is one way. I can be in InetSoft, and make calls out to Open Source. Then we're talking about things like using the open standard of a PMML. I think it's really silly, and I do see less and less of this, thank God, people debating is R better than Python better than InetSoft? These are not productive discussions, I don't think. I mean I think it's good to know which tool to use for what. I certainly think that's good. I teach a data mining class and where I expose this to my students. Know which tool saves you the most time at what point of the process. I think that's more important...
Machine Learning Applications - Machine learning applications have the power to transform companies and elevate them to new heights. InetSoft has brought its flagship BI software to the realm of machine learning by providing an integrated visual analytics interface to help end users understand the results of a machine learning model. This makes the running of ML algorithms as easy as clicking a button. Now, non data scientists can harness the power of automated data modeling to find solutions to the business challenges they face. Supervised learning is just that, learning that requires supervision. Algorithms in this category utilize your established variable prediction model and train a ML predictor variable against it to make future calculations. Unsupervised Learning Unsupervised learning, unlike supervised learning, asks machine learning to find patterns on its own. In this method, no human provides examples to be used as a base to learn from. For business applications, two types of unsupervised learning are often seen: clustering and churn. Cluster analysis is especially useful for revealing potential segmentation methods which might not have come to mind. This is done by grouping website visitors or customers into clusters. The dashboard image above is an example of churn machine learning created by InetSoft...
Machine Learning Business Application - Machine learning extracts information from data that is not apparent to even experienced business users. Data scientists normally spearhead this process using specialized data science tools such as Python and R, but Business users are most times limited to static ML output and reports. InetSoft changes that. InetSoft's data intelligence software productionalizes machine learning. It provides visual tools that directly tap into machine learning business applications. Business users can not only visually analyze machine learning results but also train machine learning models with sliced-and-diced data. When data grows beyond an experimental machine learning setup, InetSoft's native Apache Spark based platform can easily scale on demand. It can utilize an external Spark Cluster or build its own with minimal Spark expertise...