Machine Learning Technology Options
Evaluating machine learning technology options? InetSoft is an industry pioneer providing easier, more cost-effective business software solutions. Request a personalized demo.
Machine Learning Dashboard to Predict Customer Churn - Looking for a hands-on example of how machine learning dashboards can be integrated with interactive visualizations? Click on the customer churn dashboard below to watch its machine learning algorithm adjust its predictions in real time. (click filters on the dashboard and then click "train ML model")...
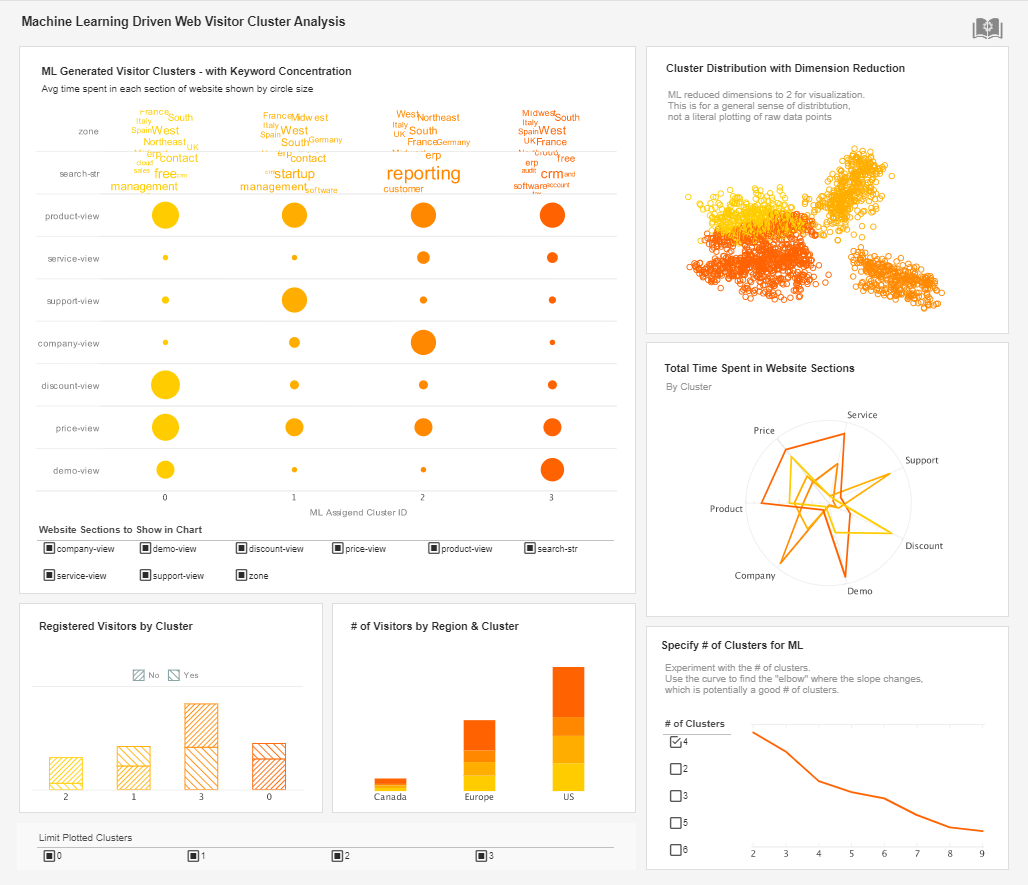 |
Click this screenshot to view a two-minute demo and get an overview of what InetSoft’s BI dashboard reporting software, Style Intelligence, can do and how easy it is to use. |
Machine Learning Is Changing Business Analytics - Thank you all for joining us today for a discussion about how machine learning is changing business analytics. We have four major points that we want to discuss today. The first one being the importance of data science and data scientist and bringing machine learning into organizations. The second one being we've all heard of the V's of big data. and we know that one is velocity, and we know that there's a lot of streaming data out there now. This is going to be a big part of organizational strategies moving forward. Point three, how an organization can keep creativity with machine learning. We have all of these different tools to choose from today, all of this different data, but we deal with regulation, we deal with documentation, we deal with productionizing code. How do we keep infusing creativity into the machine learning workflow within an organization? Then, we've also heard a lot about the citizen data scientist recently and just in general more and more people in organizations wanting to get involved with analytics and machine learning, so that's point four. Okay, so we're going to start our discussion here. Is any of this really new? Is machine learning new? Is data science new? To me this is resounding no. In fact, machine learning has been studied at least since the 1950s, maybe before. Data science you could say goes back to John Tukey's 1962 Future of Data Analysis Paper. There's a great recent paper by David Donoho out of Stanford that talks about 50 years of history of data science, and I urge you to read that. We have a link to that at the end. We're seeing machine learning in organizations now. This isn't coming out of the blue. This has a long history, and so we wanted to spend a little bit of time here. One good thing to do at first is of course to define machine learning, and that's really tricky. I think for better or for worse, in a certain sense machine learning has taken on sort of a pop culture, meaning it's just the rebranding of analytics or data mining...
Machine Learning for Marketing - When Do You Need a Data Scientist - This post is the ninth in a series discussing a machine learning use case for a mobile app provider. The link to the full case study can be found in the first post of this series: https://www.inetsoft.com/blog/machine-learning-concepts-defining-churn-predictive-metrics/ We end this series of informational posts with a question: When do I need a data scientist? To get started and to experiment with machine learning, in marketing or any business function, you do not need a data scientist. You just need to be as skilled as an Excel power user, someone who knows the data and is comfortable doing analytics. When you define the business goal, the conceptual knowledge you possess so far on machine learning from reading our series of articles should allow you to decide which class of machine learning algorithms to look at. With a proper software tool, experimenting with a small dataset is becoming more and more practical. As mentioned previous post, you can even just start with your PC. And as mentioned before, beyond the type, you don't need to understand much what the algorithm is doing. With just trial and error you can see which of the models gives the best accuracy. When you've identified the best model and its fit is over 70%, then it is worth planning on putting it into production. And that is the right time to engage with a data scientist and your IT support staff to fine tune things and take the necessary steps to productionalize the model and the subsequent business actions it calls for. Also, if you can't manage to reach a worthwhile level of accuracy, that is another time to ask a data scientist for help, and learn what possible tricks they might have up their sleeves to augment the data or seek other models. If you're ready to embark on a machine learning project, and you'd like even more handholding, we are raising our hand. Our software makes it as easy as possible to run machine learning models, and an InetSoft data scientist will be assigned to help...
#1 Ranking: Read how InetSoft was rated #1 for user adoption in G2's user survey-based index |
|
Read More |
Machine Learning and Predictive Analytics Create Data to Analyze - So we talk about machine learning and analytics, predictive analytics, the platform itself actually becomes a data creation platform, and that also adds to the different varieties of data. So it actually ends up compounding the drive to work towards the variety of the platform itself. The opportunities that it creates for using the data that comes in will undoubtedly be unlocked. It will keep growing. The varieties are never going to go in other direction. It's all is going to be increasing, and that's a great opportunity. It means different data types, script data and non SQL data will continue to increase a little. Coming back to how you actually get that data coming the other direction with SQL becoming more and more important, but in the underlying data, the variety is only going to increase. Abhishek: Larry, any thoughts you may want to add in here...
Machine Learning Use Cases That Were Impossible Before - Let's go to the other side of the business to speak about machine learning use cases that were impossible before. What if you could not just put marketing dollars in putting your brand or logo somewhere, what if you couldn't just sponsor a team or a particular venue or event but you could measure the impact and the outcome of that in real time. Thanks to computer vision and full HD video processing we are able to find your logos, your products, your offerings in real time in commercial broadcast quality and video path and to determine accurate impact metrics so that you can measure what you pay for in terms of scholarship for advertising and make that available in near real time. These are capabilities that have never been available on the market before, and this aims to revolutionize the way we do advertising impact metrics and return on advertising investment calculations. We look forward to deriving many additional visual and video base use cases that bring concrete business value to the enterprise. It's interesting to see that machine learning touches basically every aspect of a business, whether it's sales or marketing, whether it's technology, whether it's operation or whether it's finance. Machine learning is and will be everywhere. You will see. This brings us basically to the next topic about some of the popular and innovative machine learning technology and applications that are being implemented today...
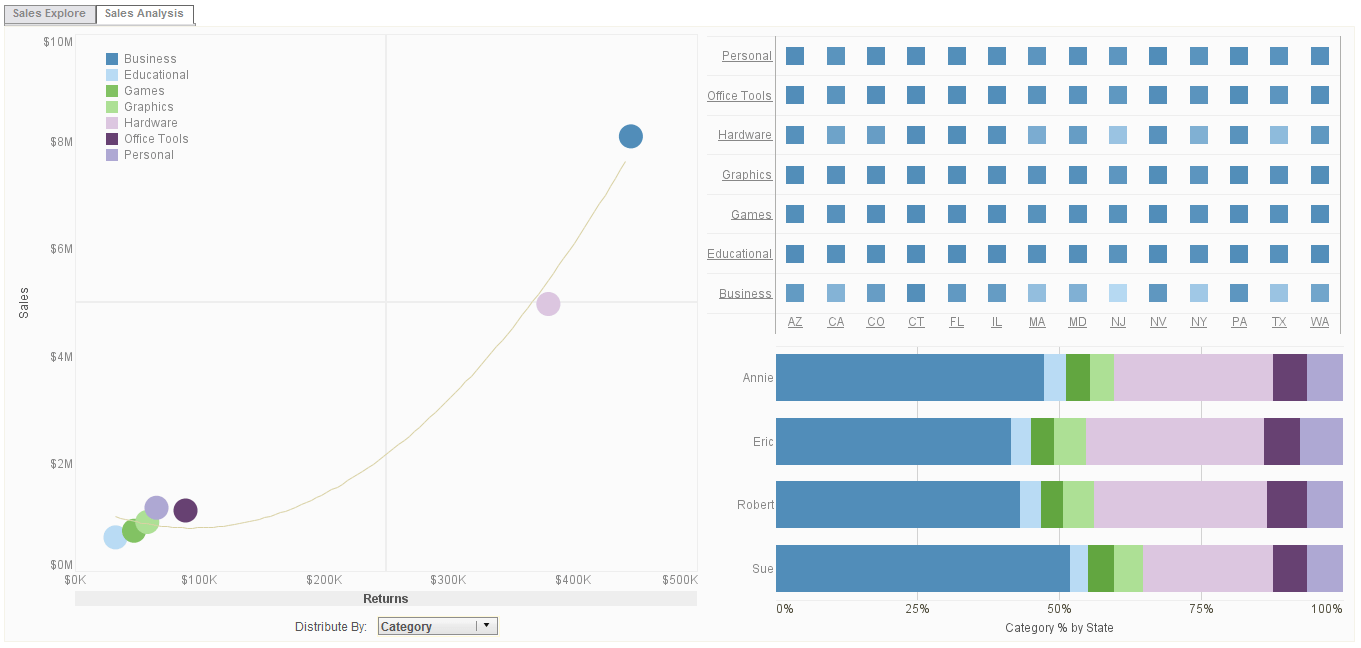 |
Click this screenshot to view a two-minute demo and get an overview of what InetSoft’s BI dashboard reporting software, Style Intelligence, can do and how easy it is to use. |
Machine Learning Utilized For Predictive Analytics - Now let's move on to machine learning which is a subset of artificial intelligence. It provides computers with the ability to learn without being explicitly programmed and utilize predictive analytics to forecast outcomes and also to assess the probability of future predictive events. Machine learning has the ability to identify risk and to identify opportunities for businesses by using cognitive computing techniques, and it supports much greater efficiency. It can understand and can respond to human sentiments and emotions as well. Machine learning has gotten beyond the capabilities of predictive analytics and beyond the capabilities of big data analytics. It also surpasses in some ways human capabilities by thinking independently and making its own evaluations and its own conclusions. If we look to the machine learning development, we see that it's accelerating very quickly, and we will discuss now the cause of this rapid advancement. One such cause is the recent explosion of big data. Data is everywhere, and it is expanding from a number of sources. You can think about the sources for text, for images, for digitized documents and for internet devices. Everything is connected right now...
Most Transformative Machine Learning Applications - Where are we witnessing the most transformative level of innovation in machine learning applications? The deep learning revolution that started in 2013 or so, this started out in the image processing space. It has since branched into video. It has branched into speech recognition and audio processing because the improvements on all new processing and quality on your mobile phones are largely driven by deep learning techniques that came into widespread use two years ago or so. They are now increasingly being applied to natural language processing with very good results. I think the next frontier for the deep learning and machine learning revolution is to also upset this status quo on reasoning and logical conclusions. This is an area where the rule based systems -- best solutions for things like natural language processing or image processing before â€" still reign supreme. We're at the next wave of deep learning based technology. It is very likely to also bring in and drive substantial improvements and assumption that help us address completing more use cases. I would definitely be reasoning as the next big frontier of what deep learning research can help deliver. Which industries will be most impacted by these developments? Looking at multiple consulting studies on this, what they all have in common is that they are from 2014, 2015 or 2016. They show a landscape of impacts all over the map. What seems to be different in how ML impacts these industries? For some it's the change in the core product or service that they are offering, like automotive manufacturers going to become self-driving car manufacturers...
Need for Mashing Up Machine Intelligence with Human Intelligence - This week Dow Jones published an interesting article about the limitations of machine learning and the need to supplement it with human intelligence. In fact, that's the inspiration of our tagline "Mashup Machine Intelligence with Human Intelligence." This article makes for good introductory reading on the topic of machine learning. It lists some common but very difficult applications of the technology such as detecting Russian spies or nude pics posted by an angry ex. For a more in-depth intro to ML for business users, see our series of posts starting with https://www.inetsoft.com/blog/machine-learning-concepts-defining-churn-predictive-metrics/ What may be surprising is the masses of humans now being employed to both assist in the training of ML models and to monitor and modify the output of ML predictions. A new industry has sprouted to offer home-based, world-dispersed workers to do some of this work. Facebook alone is having to significantly invest in staff to power its "artificial intelligence." The full article can be found here http://www.morningstar.com/news/dow-jones/us-markets/TDJNDN_20171112481/without-humans-artificial-intelligence-is-still-pretty-stupid.html As a provider of data intelligence software, our solution provides a visual approach to make it easy for non-data scientists to take advantage of machine learning. It integrates business intelligence analytics and model training in one application so you can more easily understand what the model is saying...
Predicting Customer Churn with Machine Learning - Looking for a hands-on example of how machine learning dashboards can be integrated with interactive visualizations? Click on the customer churn dashboard below to watch its machine learning algorithm adjust its predictions in real time. (click filters on the dashboard and then click "train ML model")...
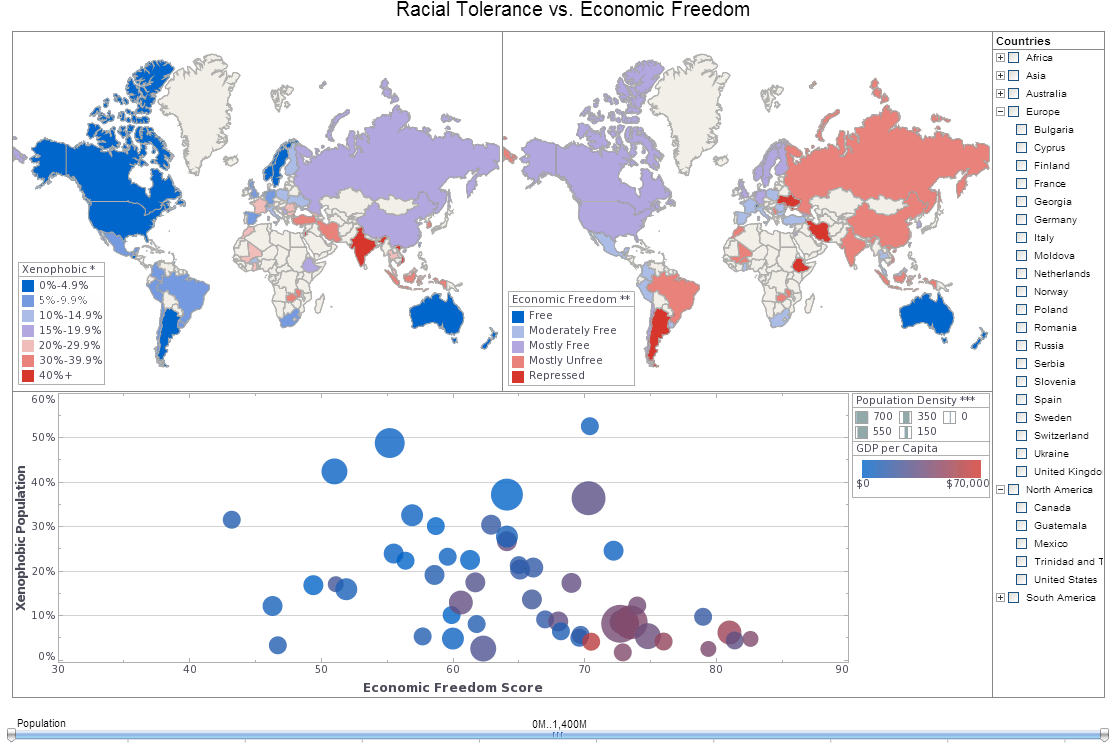 |
Click this screenshot to view a two-minute demo and get an overview of what InetSoft’s BI dashboard reporting software, Style Intelligence, can do and how easy it is to use. |
Providing Analytic Software for Machine Learning - Looking for machine learning analytic software providers? InetSoft is a pioneer in self-service analytics, and our solution lets data scientists and business users visulize model results to see which features are driving predictions. View a demo and try interactive examples...
Setting for Applying Machine Learning - Another setting for applying machine learning is the science in genetics. A lot of genetics research is done on fruit flies, and the reason is they are convenient. They breed fast, and a lot is already known about the genetics of fruit flies. The MNIST database of handwritten digits is the machine learning equivalent of fruit flies. It is publicly available. (See the Wikipedia article on the database to learn more about it https://en.wikipedia.org/wiki/MNIST_database) We can get machine learning algorithms to learn how to recognize these handwritten digits quite quickly. So it's easy to try lots of variations, and we know huge amounts about how well different machine learning methods do on MNIST. And in particular, we know that the different machine learning methods were implemented by people who believed in them, so we can rely on those results. So, for all those reasons, we are going to use MNIST as our standard task. Here is an example of how it would be used. Of all the handwritten digits in MNIST, these are ones that were correctly recognized by a neural net the first time it saw them, but there are ones were the neural net wasn't very confident about. It could be a collection of digits that look like the number 2...
Setting Up Machine Learning Solution with a Legacy System - One last question which is probably on the minds of many of our listeners is how does a legacy system affect the process of implementing machine learning solutions? That's a question that I get a lot from our installed base. InetSoft's has chosen the a route of making all machine learning solutions technically available as add-on services running either on premise or in the cloud. With our API and multiple integration points, we're able to enable a rigorous integration of machine learning capabilities into existing architectures without the need to be on the fully latest version number of every single product. I think this is something that all customers look for and appreciate because it helps them drive business value today while also embarking on longer term harmonization and then making an upgrade journey in parallel. So now I'd like to just reiterate a few of the main points as we bring this webinar to a conclusion. Artificial intelligence is enabling us to develop intelligent machines that are helping to enhance our human capabilities and further enabling enterprises to succeed. What we've seen in all the examples today is how machine learning has allowed businesses to reimagine their business processes and to reimagine all the applications of ML...
Solving Business Problems with ML - Where is machine learning being leveraged to help solve real business problems nowadays? We see a few main areas where enterprises are looking for business value and business benefits and applying machine learning to their daily operations. The first category is a top line growth. This is about serving better recommendations to customers, finding the right customers, finding the right opportunities, approaching them in the right way and upselling and cross-selling in order to drive the top line. Recommended systems is primarily a domain of natural language processing, of sales cycle, sales insight systems, as well as marketing optimizations. The second big area is to help re-imagine business processes with digital intelligence. So this is not just about redoing what we do today with ML insight but about truly rethinking the end-to-end process in a way that this leverages ML capability to deal with the routine and the optimal current cases, and this can have tremendous bottom line benefits. The third category is about employee engagement and having healthier and more satisfied employees with a more varied set of challenges during their working hours because again they can focus on the top and to the underserved cases that require new thinking and that require unstructured problem solving. This also includes automating away some of the mindless or repetitive tasks that nobody particularly enjoys about their daily activities today...