What Is Operational Data Architecture?
Data architecture is the foundation of any organization's data management strategy, and it plays a crucial role in ensuring that data is accurate, consistent, and accessible. In recent years, there has been a significant shift in focus towards managing data for analytics, while operational data has often been considered as mere source data for analytics.
However, operational data is much more than just source data for analytics. It is the data that is used to run the business on a day-to-day basis. Ignoring operational data as a critical part of data management architecture can lead to significant challenges in managing data sprawl, data silos, and data disparity.
Operational data architecture is the design and organization of the data that is used to run an organization's operations. It is concerned with how operational data is captured, stored, managed, processed, and accessed to support the daily operations of an organization. In this article, we will explore the nature of operational systems, the challenges of operational data architecture, and how to address these challenges.
#1 Ranking: Read how InetSoft was rated #1 for user adoption in G2's user survey-based index |
|
Read More |
The Nature of Operational Systems
Operational systems are the systems that are used to run the day-to-day operations of an organization. These systems have evolved significantly over the years, from primarily transactional systems to encompassing transactions, workflow automation, process automation, commercial IoT, and industrial IoT. Each of these systems poses key architectural questions that need to be addressed by data teams.
Transactional Systems
Transactional systems record business interactions as they occur in the day-to-day operation of the business. These systems capture data as a historical record and audit trail of business activity. Transactions may be recorded in real-time or with some latency. Transactional data typically flows to analytics systems as source data for data warehousing and data lakes, often with the latency inherent in batch processing. Data architecture needs to rethink how to capture and process transactional data in real-time to support real-time decision-making.
Workflow Automation
Workflow automation streamlines business processes, coordinating actions across systems, data, and teams. Workflow systems support workflow and document configuration, task management, document flow, authorization and signatures, and policy compliance tracking. These systems collect and store data in the form of documents as well as records of document routing and processing. Data architecture needs to determine whether documents or document flow records should flow to a data lake to support operational and analytical needs.
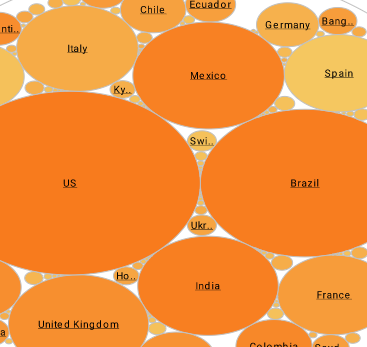 |
“Flexible product with great training and support. The product has been very useful for quickly creating dashboards and data views. Support and training has always been available to us and quick to respond.
- George R, Information Technology Specialist at Sonepar USA
|
Manufacturing Automation
Manufacturing automation systems integrate machines, software, and data to optimize manufacturing processes. These systems rely heavily on real-time and very low latency data. Machines receive data to control their operations and collect data to monitor conditions and behaviors. Data architecture needs to consider whether real-time machine data should also flow to a warehouse or data lake as a historical record of machine operations and performance.
Commercial IoT Systems
Commercial IoT systems integrate software, networked devices, and real-time data to automate management processes for commercial buildings such as office buildings, stores, shopping malls, hotels, etc. These systems monitor and adjust environmental conditions such as temperature and air quality, economize utility costs and energy consumption, manage and monitor building access, and provide other smart building capabilities. Data architecture needs to determine whether real-time data from these systems should also flow to a data lake to record the history of smart building operations.
Industrial IoT Systems
Industrial IoT (IIoT) systems interconnect sensors, instruments, machines, devices, and computer applications such as manufacturing and energy management. These interconnected components collect and exchange data to achieve productivity and efficiency gains. These systems are heavily reliant on real-time data. Data architecture needs to determine whether real-time data from IIoT systems should also flow to a data lake to record the history of industrial operations.
The Challenges of Operational Systems
Operational systems include legacy systems and an increasing proportion of purchased applications (ERP, SaaS, etc.), each with unique semantics, Interoperability Between Analytic and Operational Data Architectures
In addition to the issues of data sharing and data integration among operational systems, there is also the challenge of interoperability between analytic and operational data architectures. The traditional focus of data architecture on managing data for analytics has resulted in a disconnect between operational and analytic data architectures. The data models, data structures, and data definitions used in operational systems are often different from those used in analytic systems, making it difficult to integrate data from operational systems into analytic systems.
This disconnect has led to the development of data warehouses and data lakes as the primary means of integrating operational data with analytic data. However, data warehouses and data lakes are often designed with a focus on analytics rather than operational data management, which can result in suboptimal performance and limited functionality when it comes to managing operational data.
Operational Data Architecture: A New Approach
To address the challenges of managing operational data, a new approach to data architecture is needed that puts operational data front and center. Operational data architecture is a holistic approach to data architecture that incorporates the unique characteristics of operational systems and data, and focuses on managing operational data as a critical part of data management architecture.
Operational data architecture involves a range of activities, including:
- Identifying and understanding the unique characteristics of operational systems and data.
- Developing data models, data structures, and data definitions that are specific to operational data.
- Implementing data management processes and tools that are tailored to the requirements of operational data.
- Integrating operational data with analytic data in a way that preserves the integrity and consistency of both data sets.
- Ensuring that data quality and data governance practices are applied consistently across all data sets, including operational data.
The key principles of operational data architecture include:
- Understanding the nature and characteristics of operational data, and recognizing that it is a critical part of data management architecture.
- Incorporating operational data into data architecture and data management processes, rather than treating it as an afterthought.
- Recognizing that operational data has unique requirements that may differ from those of analytic data, and tailoring data management processes and tools accordingly.
- Ensuring that data quality and data governance practices are applied consistently across all data sets, including operational data.
- Integrating operational data with analytic data in a way that preserves the integrity and consistency of both data sets.
Benefits of Operational Data Architecture
Adopting an operational data architecture approach can bring a range of benefits to organizations, including:
- Improved data quality: By incorporating operational data into data architecture and data management processes, organizations can ensure that data quality and data governance practices are applied consistently across all data sets.
- Better data integration: By tailoring data management processes and tools to the requirements of operational data, organizations can improve the integration of operational data with analytic data.
- Reduced technical debt: By addressing the challenges of managing operational data, organizations can reduce the technical debt associated with legacy systems and proprietary applications.
- Faster time-to-insight: By integrating operational data with analytic data in a way that preserves the integrity and consistency of both data sets, organizations can accelerate the time-to-insight for analytics and business intelligence.
- Improved business outcomes: By leveraging operational data to improve operational efficiency and effectiveness, organizations can achieve better business outcomes and drive growth.